Mitigating Risk in a Three-Sided Marketplace: A Conversation with Trupti Natu and Neel Mouleeswaran on the Uber Eats Risk Team
March 12, 2019 / Global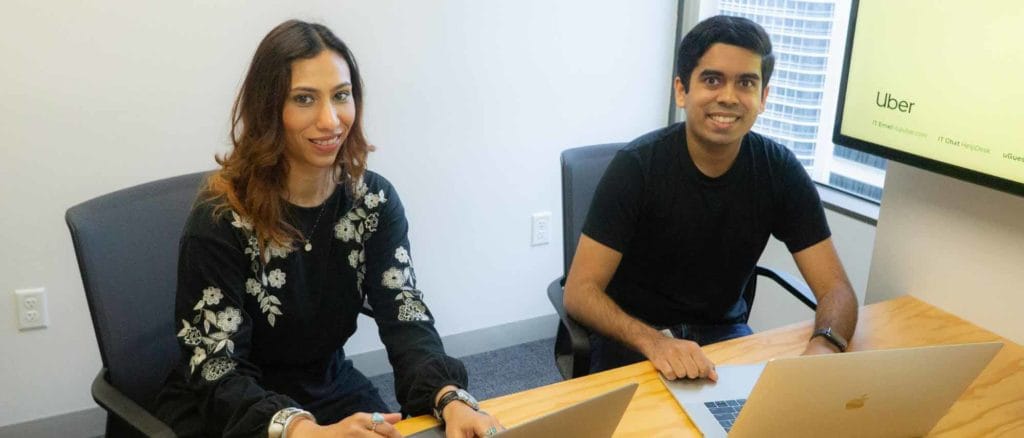
Under the hood of the core Uber Eats experience—tap a button, place an order—is a three-sided marketplace of eaters, delivery-partners, and restaurant-partners connected by a complex network of features and services that facilitate the seamless delivery of your favorite meals.
This three-sided marketplace relies on smooth, secure transactions between users on our payment platform. With over eight ways to pay using the Uber Eats app, it is critical that our platform facilitates quick, frictionless interactions while ensuring the integrity of user payments and earnings.
Turning this vision into a scalable reality is the Uber Eats Risk Analysis team, a group of data scientists, engineers, and strategists responsible for mitigating risk on our payments platform through technology, research, and policy. In addition to designing and building complex back-end systems to validate payments integrity, this team develops tools to thwart risk in real time by identifying suspicious activity and freezing bad actors in their tracks.
To better understand the world of risk analysis at Uber, we sat down with Trupti Natu, a risk strategy manager, and Neel Mouleeswaran, a risk engineer, to discuss how they got interested in risk, why they came to Uber, and what is most rewarding (and challenging) about minimizing risk for a three-sided marketplace:
How did you first get interested in risk engineering and risk analytics?
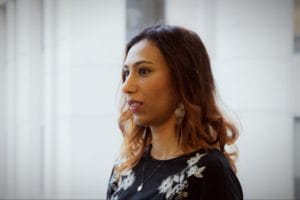
Trupti: My background is in FinTech: payments, credit cards, and gift cards. Payment, in general, goes hand in hand with risk. I’ve always partnered with risk teams, even when I was in FinTech. When Uber approached me for this role, it seemed like an exciting opportunity to challenge myself and expand my career space. Ever since I started, it’s been an amazing ride.
Neel: My background is in software engineering, mainly in back-end systems. When I was in college, I had the opportunity to intern at Uber on the fraud team, which was an awesome experience. While on the fraud team, I had my first exposure to working in risk. Along with getting to work on cool and challenging projects, what also interested me about risk analysis was getting to think about how fraudsters find vulnerabilities and how we can build solutions to continuously stay one step ahead of them.
What drew you to working at Uber?
Trupti: Even before joining Uber, I was a frequent user of our rider app. A few years ago, I moved from Manhattan (where most people don’t own a car) to Seattle (where many people do), and Uber made it possible for me to get around without buying a car. Transportation is very different when you move from New York City to really anywhere else. Uber gave me additional transportation flexibility when I moved.
Since I’d been an Uber loyalist for awhile and was in love with the product, when the opportunity came around to join the Risk team, I didn’t think twice. I was looking for something new, something more challenging at a company where I knew I could make an impact. In many ways, Uber—especially Uber Eats—feels like a startup nestled in a larger company. This creates an environment where you can make a mark and also have an ecosystem that supports your bold ideas.
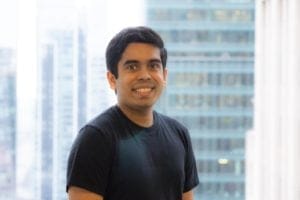
Neel: I became interested in working at Uber after creating a hackathon project in college where we used the Uber API. One of the engineers who helped me get set up told me about his experience working at Uber. I read more about it later and I was very impressed at how much engineering work is going on behind the scenes on the Uber platform. Uber is also a very global company and I was excited to get the chance to work on software that would positively impact people all around the world.
What is risk management? What types of technologies and strategies do you use to mitigate risk on the Uber platform?
Trupti: Risk management is a broad bucket. What we deal with on the Uber Eats side of things is primarily eliminating or stopping fraudsters from abusing our platform to defraud Uber or our customers. It boils down to tactical things like reducing losses from fraud and improving the experience for our customers. These savings in turn become investments that fund our big, bold bets and make sure we continually improve our product. In many ways, it’s like a flywheel effect.
Neel: Our solutions try to maintain the balance of reducing fraud loss without compromising the user experience by adding unnecessary friction. We deploy fraud rules and machine learning models by collaborating with analytics and data science, building on top of platform services. This risk engine helps us run fraud assessments in real time and at a very large scale. Risk management even extends to the app. We can lower risk for our riders and eaters by, for example, applying risk decisioning to what kind of payments are safer to use in certain situations.
Trupti: While much of risk is about stopping fraudsters, many of our projects revolve around preventing fraud in the first place. We base these decisions on data that is on our platform, which helps us make precise, data-driven decisions so that we can facilitate the best experience possible for our customers and partners.
Can you give an example of a recent project that combined both risk management analysis and engineering?
Trupti: A recent collaboration between analytics and engineering was our non-perishables tagging project. Risk holds the most features [data attributes] amongst all Uber teams, making our roles very multi-layered from a data science perspective. For instance, one of the attributes critical to fraud detection, as you can imagine, is how to identify an non-perishable item, which is based on manual labeling menu items. Because of the complex nature of defining and categorizing these items, there were some discrepancies in identifying this manually tagged non-perishable dataset.
With non-perishables, we wanted to make sure the decisions we made to categorize it are based on accurate data. We are a technology company and so we decided to use machine learning (ML)-based models to identify non-perishable items. This was just one use case for the new ML system we built, but it can further be used to classify perishables as opposed to non-perishables and risky as opposed to not-so-risky menu items.
Neel: Part of the ML solution was to improve the precision of the tagging, but also to enable automatic tagging as soon as any restaurant onboards or a new menu item is added. We think this could expand to other areas, too, perhaps also for menu items with specific allergens or other nutritional information that might be useful. Anything that we could infer about the item from the model could be used in assessing risk, and potentially applicable to other types of checks and detections.
What makes risk management so interesting on the Uber Eats platform?
Trupti: Uber Eats’ three-sided marketplace of eaters, delivery-partners, and restaurant-partners adds an extra layer of complexity compared to the two-sided marketplace of Uber’s rideshare business. On top of that, Uber Eats is a real-time marketplace. Since we are customer and partner-obsessed, any action that we take in order to stop fraudulent behavior could also potentially impact a well-meaning customer, for instance, in the case of a false positive.
For example, say you’re a brand-new user, and you’ve just placed your second Uber Eats order. You’re in Hong Kong this weekend on a business trip, but you registered your account in the U.S. and added a credit card from the U.K. These are typically signals that are associated with a fraudster, and so chances are you might experience some kind of friction because we want to make sure your account or card data wasn’t compromised and it’s really you and not a bad actor. In such cases, we want to minimize friction while also maintaining the integrity of user transactions. It can be difficult to be precise when you are operating in a real-time marketplace and there are so many different policies and transaction requirements for each region you operate in, as is the case for Uber.
All that said, being global and real-time is something many users love about Uber Eats. They can open the app and order anywhere that we operate. However, that does bring an extra level of complexity to our work as risk managers, as we are essentially the gatekeepers of financial transactions on the platform. At the end of the day, we have to pay our driver-partners and restaurant-partners irrespective of whether we get paid by our customers or get defrauded by fraudsters. We have to make sure the economics make sense and are fair to our partners.
Neel: On the engineering side, Uber Eats’ three-sided marketplaces introduces even more complexity. With Uber Eats, you can have multiple orders in flight at the same time, too. We have to develop a good understanding of an eater’s risk profile and automatically take decisions at different phases of the user lifecycle to prevent abuse.While we have built risk infrastructure to address the different ways in which we can get defrauded, we also have to think about how to make our systems extensible for new use cases.
What are some of the solutions your team has built to detect and mitigate risk on the Uber Eats platform?
Trupti: Primarily, our engineering work focuses on building technologies to detect fraud and then take steps to mitigate it. For instance, Mastermind is a rules engine that is the brain behind all risk rules decision making at Uber. Risk analysts and engineers add policy-based, modus operandi-based and model-based rules on this platform to keep users on the Uber platform safe from fraudsters and bad actors.
We implemented authorization holds to help protect against insufficient funds for an Eats order r. The system holds the order amount upfront on the eater’s payment method and then processes it at delivery time. To optimize user experience, the implementation has to handle scenarios such as repeated cancellations and refunds.
Neel: We also built Sherlock, a manual review tool. Sherlock helps streamline the case review process by aggregating fraudulent cases into queues and enabling city teams to provide feedback.
Like many teams at Uber, feature engineering is an ongoing effort that improves the precision of our rules and models. We work with analytics and data science groups to determine a mix of real-time and offline features that will get the best fraud signal for a given eater, order, and payment method. We have to develop a good understanding of the services and data sources within Uber in order to build new features. Additionally, the feature pipelines need to withstand the scale of Uber’s growth without sacrificing the freshness or accuracy of the features they produce.
What would you say is the most rewarding part of working on the risk management team?
Trupti: I love my job for two reasons. The first reason is the cross-functional, collaborative nature of my work. I love that risk is very horizontal. I manage global risk for Uber Eats, meaning, I work with all regions and across teams (eater, delivery-partner, restaurant-partner, payments, etc.) within Eats. Sometimes, I get this question from talking to people that we closely work with. They ask, “how do you connect the dots?” Because in this role I am so front and center and involved with almost every product launch or update. I have a vantage point such that I get an end-to-end view of a product’s lifecycle that helps piece things together.
The second reason I love my job is the people I get to work with. Neel is a great example, we work so well together, I couldn’t have asked for a better engineer to collaborate with me. Everyone on the Risk and Uber Eats teams that I work with are just incredibly talented and yet very humble and approachable. There is so much to learn from each of them. I find myself very lucky because it’s rare to have such a good blend of talents and skill sets.
Neel: I find Uber to be very collaborative. In addition to Engineering, I also get to work with other teams such as Data Science, Analytics, and Product. I like getting to interact with these other teams and functions, because it gives me the the chance to learn something new nearly every day. Since I joined in 2016, I’ve seen Uber Eats grow rapidly in the last few years into a multi-billion dollar business. Being on the frontlines as we’re growing the business at scale is rewarding.
What drives you outside of your work at Uber?
Trupti: I’m very involved with my alumni chapter at Carnegie-Mellon University. I love event planning and mentoring students and recent alums regarding career advice. Also, I love to work out—yoga, running, swimming, triathlons (aqua-thons)—you name it. Currently working on perfecting my handstand push-up.
Neel: I enjoy running and playing basketball. There’s a group of us that play together after work. I also play the sitar in my free time–music is something I’ve been doing since I was really little. Particularly on a team like Risk, it’s important to lead a balanced life and not let the day-to-day work consume your downtime. In this way, sports and music helps me mitigate “risk” (as in risk engineering).
If building risk management systems for Uber Eats and other product teams interests you, consider applying for a role with Uber.
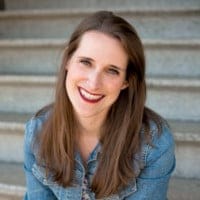
Molly Vorwerck
Molly Vorwerck is the Eng Blog Lead and a senior program manager on Uber's Tech Brand Team, responsible for overseeing the company's technical narratives and content production. In a previous life, Molly worked in journalism and public relations. In her spare time, she enjoys scouring record stores for Elvis Presley records, reading and writing fiction, and watching The Great British Baking Show.
Posted by Molly Vorwerck
Related articles
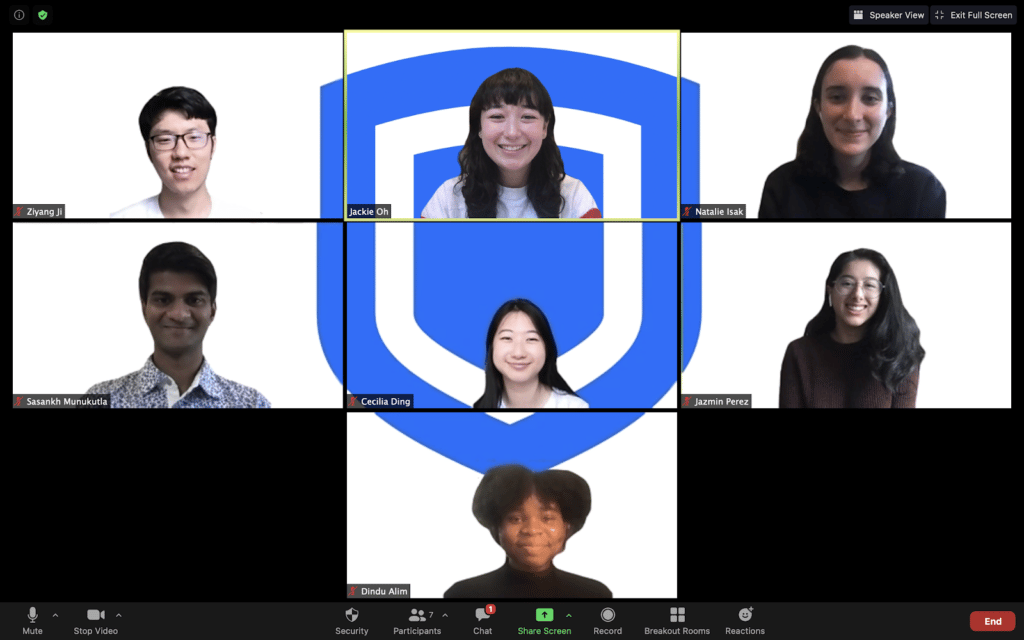
Meet the 2020 Safety Engineering Interns: COVID Edition
October 29, 2020 / Global
Most popular
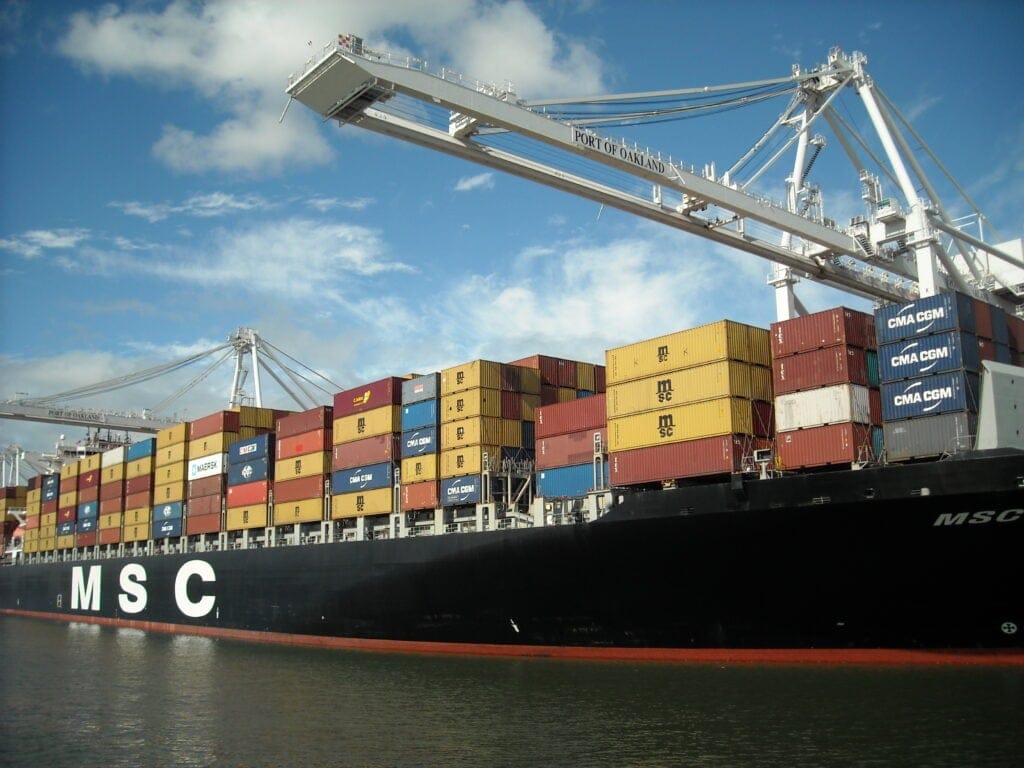
Migrating Uber’s Compute Platform to Kubernetes: A Technical Journey
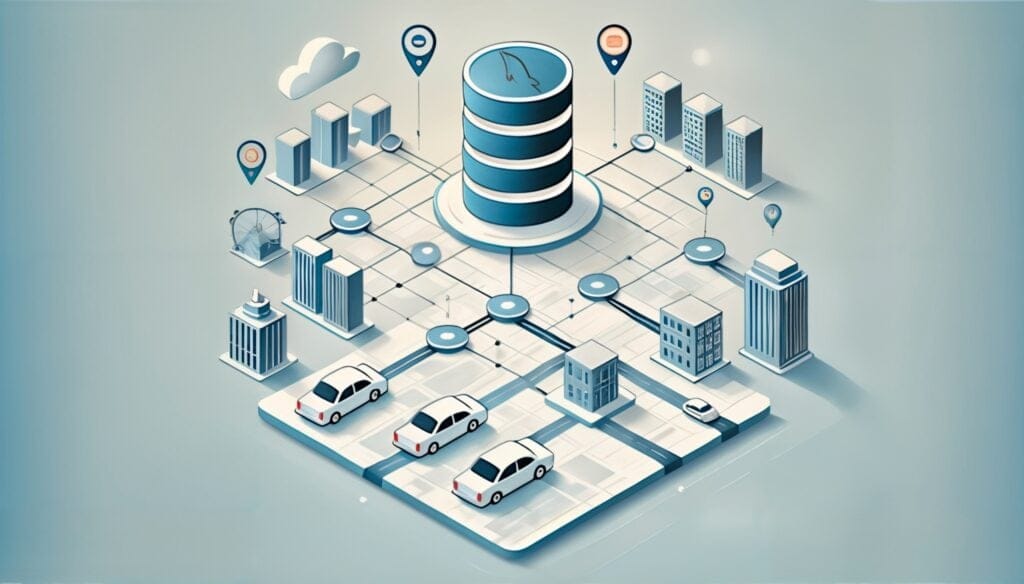
MySQL At Uber
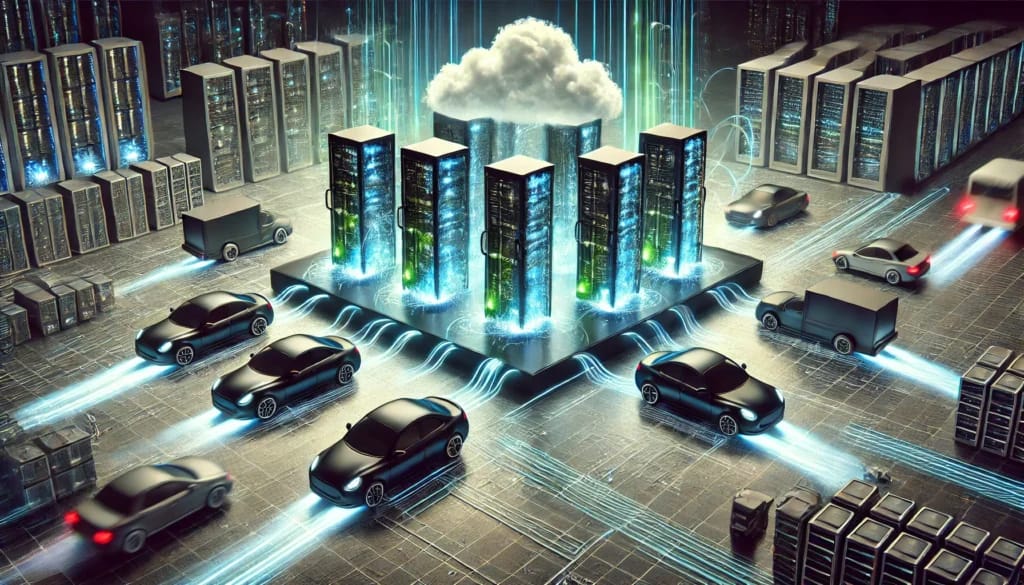
Adopting Arm at Scale: Bootstrapping Infrastructure
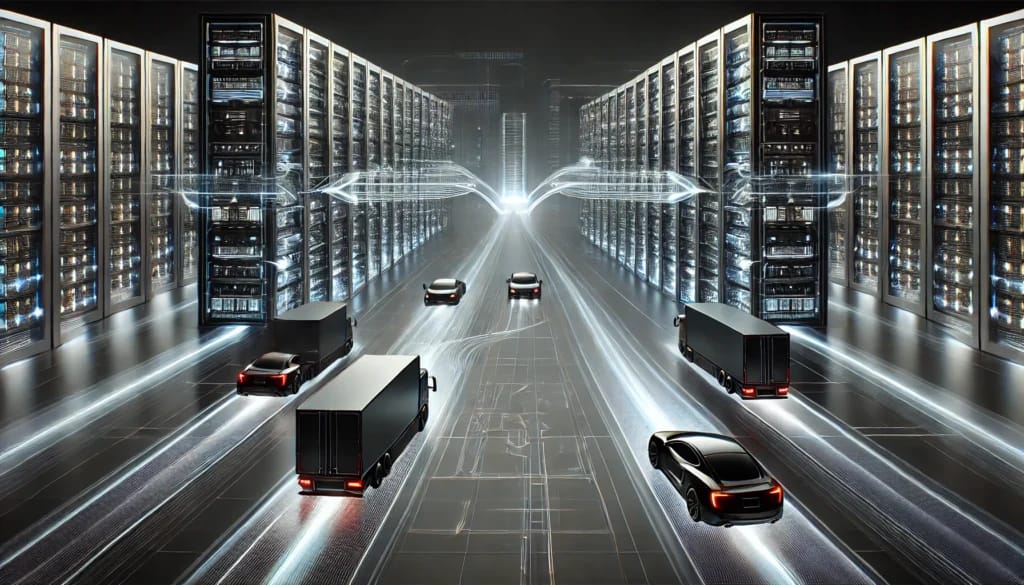