How Uber Engineering Evaluated JSON Encoding and Compression Algorithms to Put the Squeeze on Trip Data
February 16, 2016 / Global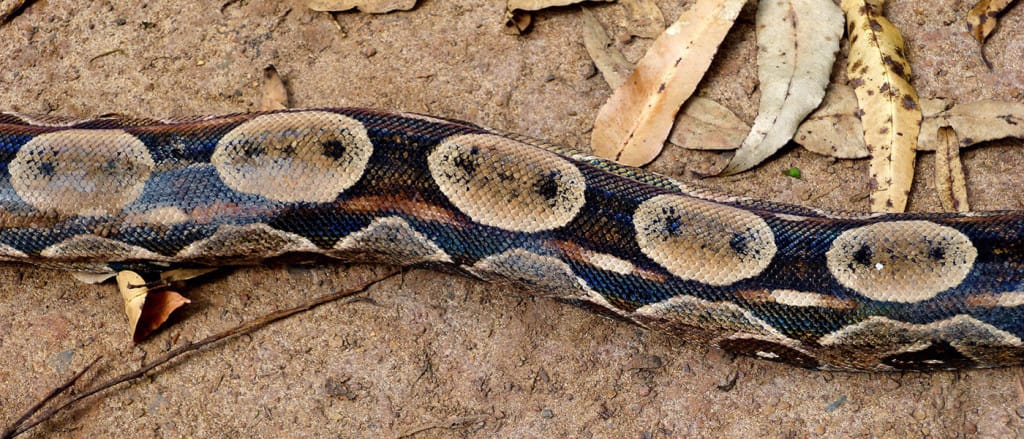
Imagine you have to store data whose massive influx increases by the hour. Your first priority, after making sure you can easily add storage capacity, is to try and reduce the data’s footprint to save space. But how? This is the story of Uber Engineering’s comprehensive encoding protocol and compression algorithm test and how this discipline saved space in our Schemaless datastores.
As of early 2016, many millions of trips flow through Uber’s platform every day across 400+ cities in over 60 countries on 6 continents. Between services, trip data is often passed around as JSON blobs, which typically take up 20 kilobytes (KB) a piece. Eventually, trip data is stored in Mezzanine, Uber’s Schemaless-backed datastore, for further processing, billing, and analytics. Schemaless is inherently append-only storage, so data piles up.
Let’s do the math: each million trips at 20 KB yields 20 gigabytes (GB) of trip data per day. Schemaless stores its data across many physical hosts. If Uber were not a hypergrowth company and trip growth instead expanded linearly, a single disk of 1 terabyte (TB) would last just 51 days. Subtract from that ~40% of space used by system components, and you’re down to 30 days per host. Thus an installation of 32 TB lasts < 3 years for 1 million trips, < 1 year for 3 million trips, and < 4 months for 10 million trips—that is, if you store raw JSON.
Since JSON data lends itself very well to compression, we were convinced we could find an algorithm that could squeeze the data without sacrificing performance. Reclaiming several KB per trip across hundreds of millions of trips during the year would save us lots of space and give us room to grow.
A Matter of Protocol
JSON bridges the gap between human readable and machine parsable via a more compact syntax than SGML and XML, but it is still ultimately ASCII. The first natural step when optimizing for space is to use a binary encoding instead, and then put a compression algorithm on top.
Encoding protocols fall into two major categories: protocols using an IDL and those that don’t. IDL-based encodings require schema definitions. They offer peace of mind with respect to data format and validation for consumers while sacrificing flexibility in the schema’s evolution. Non-IDL-based encodings are typically generic object serialization specifications, which define a compact format on top of a fixed type system. They provide a flexible serialization mechanism but only give basic validation on types. We evaluated three IDL based encoding protocols and seven non-IDL based encodings:
Encoding Protocol | Schema-based (IDL) | |
1 | Thrift | Yes |
2 | Protocol Buffers | Yes |
3 | Avro | Yes |
4 | JSON | No |
5 | UJSON | No |
6 | CBOR | No |
7 | BSON | No |
8 | MessagePack | No |
9 | Marshal | No |
10 | Pickle¹ | No |
For compression, we put three lossless and widely accepted libraries to the test:
Snappy aims to provide high speeds and reasonable compression. BZ2 trades speed for better compression, and zlib falls somewhere between them.
Testing
Our goal was to find the combination of encoding protocol and compression algorithm with the most compact result at the highest speed. We tested encoding protocol and compression algorithm combinations on 2,219 pseudorandom anonymized trips from Uber New York City (put in a text file as JSON). We wrote a test script in Python to benchmark each option (IDL files were handcrafted from trip JSON data for Thrift, Protocol Buffers, and Avro). Then, the script was put to work. Looping through all, the script measured time spent encoding/decoding, compressing/inflating, and the gain or loss in size. Here it is in pseudocode:
Comparing Results by Size and Speed
For size, the combination Protocol Buffers (PROTOBUF) compressed with zlib was just slightly better than Thrift with BZ2, squeezing data to just above 8% of its uncompressed JSON equivalent. Disturbingly, storing pickled data was worse than just persisting raw JSON.
For speed, Thrift won the race by spending only 1548 ms: 23% of the 6535 ms it takes to use JSON with the native C++ backed implementation, and vice versa. The native Python Avro implementation, on the other hand, ran at 211,540 ms: more than 32 times slower than the native JSON encoder. There is a fastavro implementation, which claims to be an order of magnitude better, but it is not feature complete and thus wasn’t tested.
The Verdict
The tradeoffs of each encoding and compressing option can be evaluated against each other in a scatter diagram. The pareto front, shown as a red line on the graph, potentially gives us the most optimal solutions:
Essentially, the bottom left corner is what we were aiming for: small size and a short time to encode and decode.
Key conclusions:
- Simply compressing JSON with zlib would yield a reasonable tradeoff in size and speed. The result would be just a little bigger, but execution was much faster than using BZ2 on JSON.
- Going with IDL-based protocols, Thrift and Protocol Buffers compressed with zlib or Snappy would give us the best gain in size and/or speed.
Since JSON compressed with zlib was in fact a good candidate, we decided to measure up the remaining contenders against that baseline. So we immediately ruled out any option that fell below JSON/zlib in either speed or size. We were left with the following shortlist:
Encoder | Encode (ms) | Decode (ms) | Size (bytes) | Size Factor | Speed Factor |
PROTOBUF zlib | 2158 | 925 | 10,885,018 | 46% | 34% |
THRIFT bz2 | 5531 | 2003 | 11,178,018 | 47% | 82% |
PROTOBUF bz2 | 5111 | 1738 | 12,023,408 | 51% | 75% |
THRIFT zlib | 1817 | 1147 | 12,451,285 | 53% | 32% |
PROTOBUF Snappy | 1224 | 790 | 14,694,130 | 62% | 22% |
CBOR zlib | 2573 | 2611 | 18,290,630 | 78% | 57% |
MESSAGEPACK zlib | 4231 | 715 | 18,312,106 | 78% | 54% |
MARSHAL zlib | 2095 | 1416 | 18,567,296 | 79% | 38% |
THRIFT Snappy | 628 | 1011 | 19,003,267 | 81% | 18% |
UJSON zlib | 2956 | 1165 | 19,917,716 | 85% | 45% |
JSON zlib | 5561 | 3586 | 23,560,699 | 100% | 100% |
Prior to Fall 2014, JSON structures passed between Uber’s services were not under strict schema enforcement. Using an IDL-based encoding protocol would require us to define IDL schemas and enforce them in Schemaless. This reduced the original list to the following contenders:
Encoder | Encode (ms) | Decode (ms) | Size (bytes) | Size Factor | Speed Factor |
MESSAGEPACK zlib | 4231 | 715 | 18,312,106 | 78% | 54% |
CBOR zlib | 2573 | 2611 | 18,290,630 | 78% | 57% |
MARSHAL zlib | 2095 | 1416 | 18,567,296 | 79% | 38% |
UJSON zlib | 2956 | 1165 | 19,917,716 | 85% | 45% |
JSON zlib | 5561 | 3586 | 23,560,699 | 100% | 100% |
Marshal, being Python only, was out by default. JSON with zlib was bigger and slower than the rest of the pack, and while that left UJSON as the fastest candidate, the size was still a bit larger than CBOR and MessagePack. The final round of evaluation was between MessagePack and CBOR. CBOR proved to be slower, so when the scripts and judging ended, MessagePack with zlib was left standing.
MessagePack/zlib is a much better choice than using the Python JSON encoder with no compression. While encoding is slower, decoding is much faster, and the relative size is an order of magnitude better:
Encoder | Encode (ms) | Decode (ms) | Size (bytes) | Size Factor | Speed Factor |
JSON | 3260 | 3275 | 132,852,837 | 564% | 71% |
MESSAGEPACK zlib | 4231 | 715 | 18,312,106 | 78% | 54% |
What We Learned
There is a plethora of encoding protocols out there, and plentiful compression algorithms as well. We settled on MessagePack with zlib. We felt this was the best choice for our Python-based, sharded datastore with no strict schema enforcement (Schemaless). We only discovered this combination because we took a disciplined approach to test a wide range of protocols and algorithm combinations on real data and production hardware. First lesson learned: when in doubt, invest in benchmarking.
How much did we save in this instance? Let’s do the math again, this time reducing 20 KB by 86% to get 2,822 bytes, the size gain yielded by MessagePack+zlib over raw JSON. Multiply by a million trips and the storage space only increases by just under 3 GB, compared to 20 GB without compression. A 1 TB disk will now last almost a year (347 days), compared to a month (30 days) without compression. Assuming a Schemaless installation with 32 TB capacity and linear growth, we now have enough space to last over 30 years compared to just under 1 year, thanks to putting the squeeze on the data.
Encoding and compressing data is a smart move, and like a three star Michelin restaurant, it’s worth the journey to get there. Not only does it save space; it also significantly reduces the amount of time spent processing data. In everyday operations, this translates directly to hardware, which does not have to be bought, provisioned, and configured.
¹ Marshal and Pickle are Python only. In general, it’s ill-advised to tie the persistent representation of data to any particular language, but we decided to include them in the test for reference nevertheless.
Kåre Kjelstrøm is a software engineer and engineering manager on the Schemaless project and works at the Uber Engineering office in Aarhus, Denmark.
Photo Credits for Header: “Boa constrictor, Atlantic forest, northeastern Bahia, Brazil” by Alex Popovkin, Bahia, Brazil licensed under CC-BY 2.0. Image cropped for header dimensions and color corrected.
Header Explanation: Boa constrictors immobilize and incapacitate prey by putting the squeeze on them with pressures of over 100 kPa (the same order of magnitude of a champagne bottle popping.)
Like what you’re reading? Sign up for our newsletter for updates from the Uber Engineering blog.
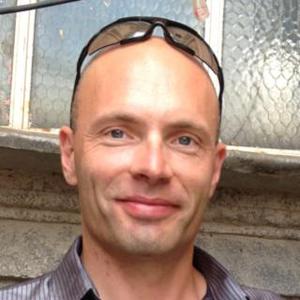
Kåre Kjelstrøm
Kare is a senior engineering manager on Uber's Core Infrastructure team.
Posted by Kåre Kjelstrøm
Related articles
Most popular
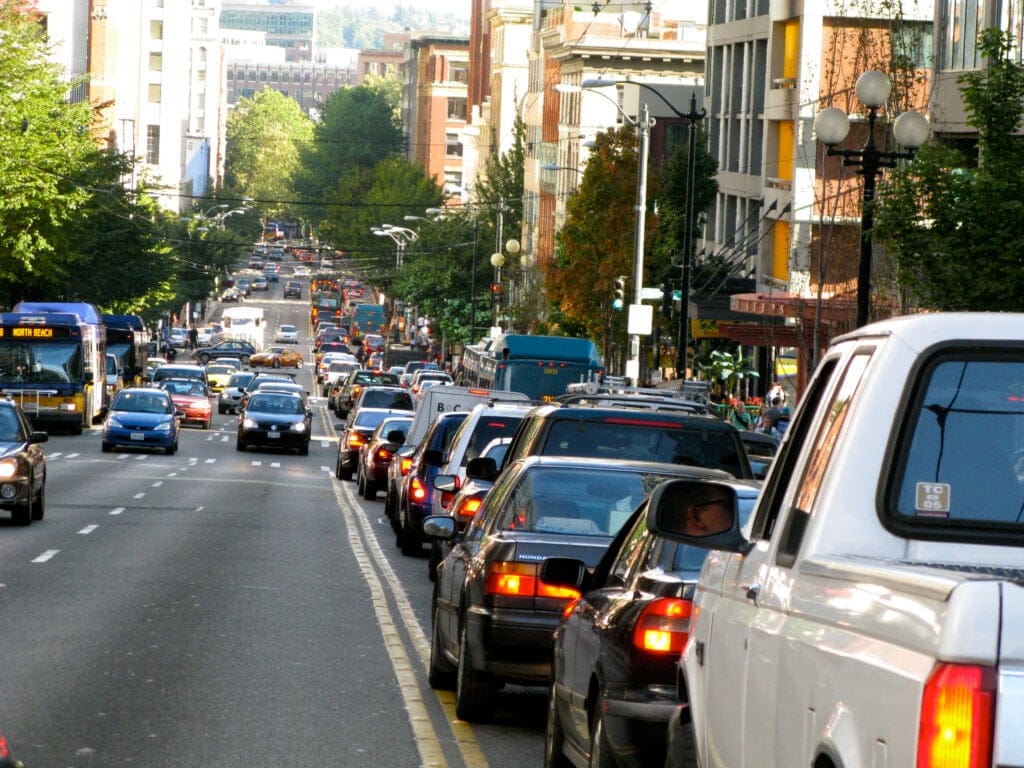
Presto® Express: Speeding up Query Processing with Minimal Resources
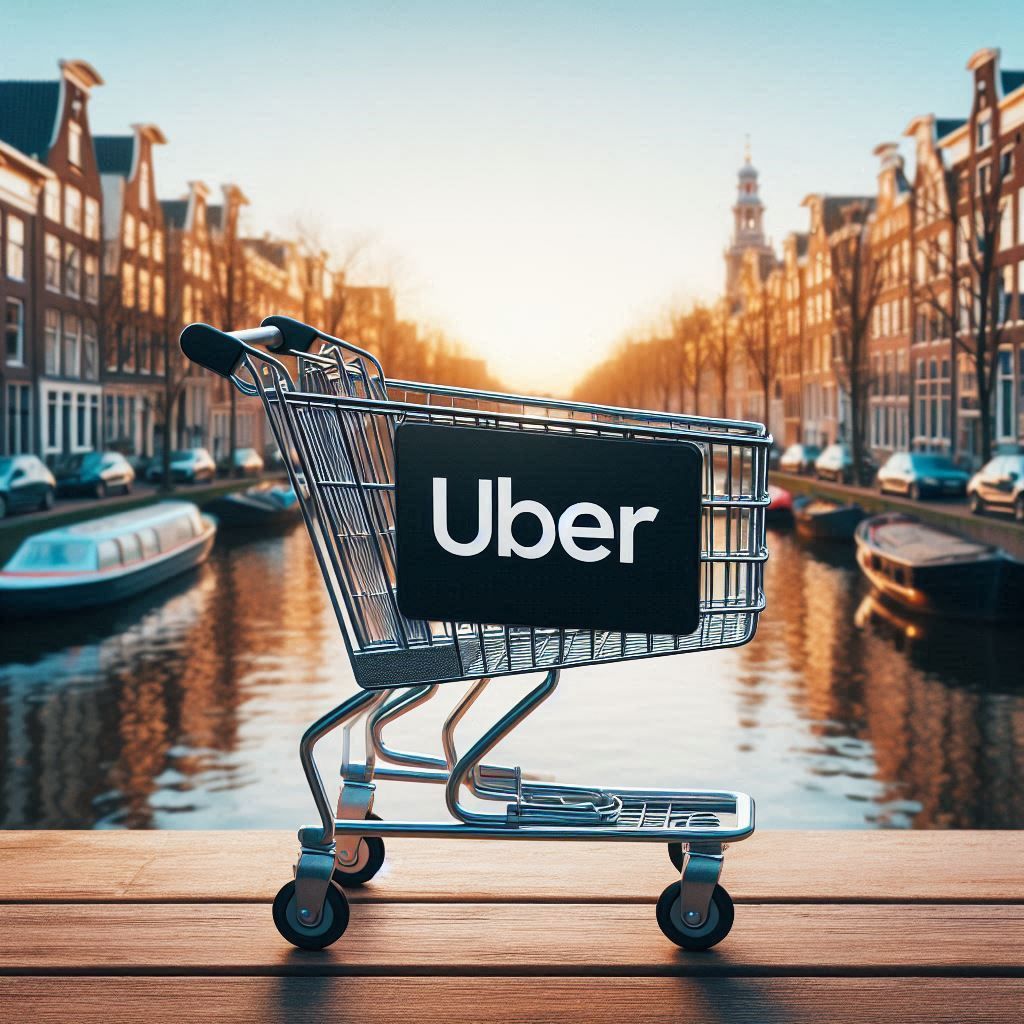
Unified Checkout: Streamlining Uber’s Payment Ecosystem
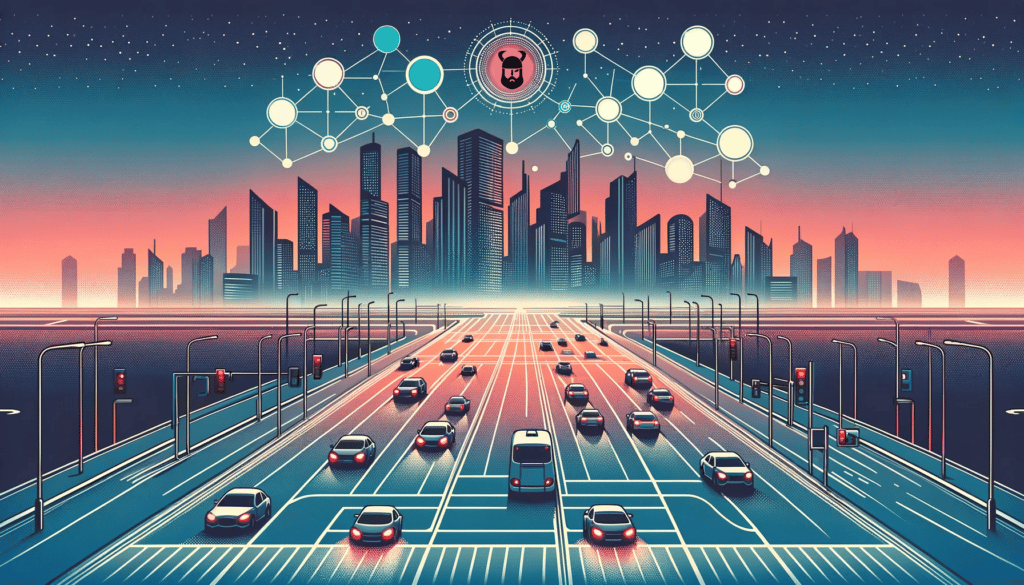
The Accounter: Scaling Operational Throughput on Uber’s Stateful Platform
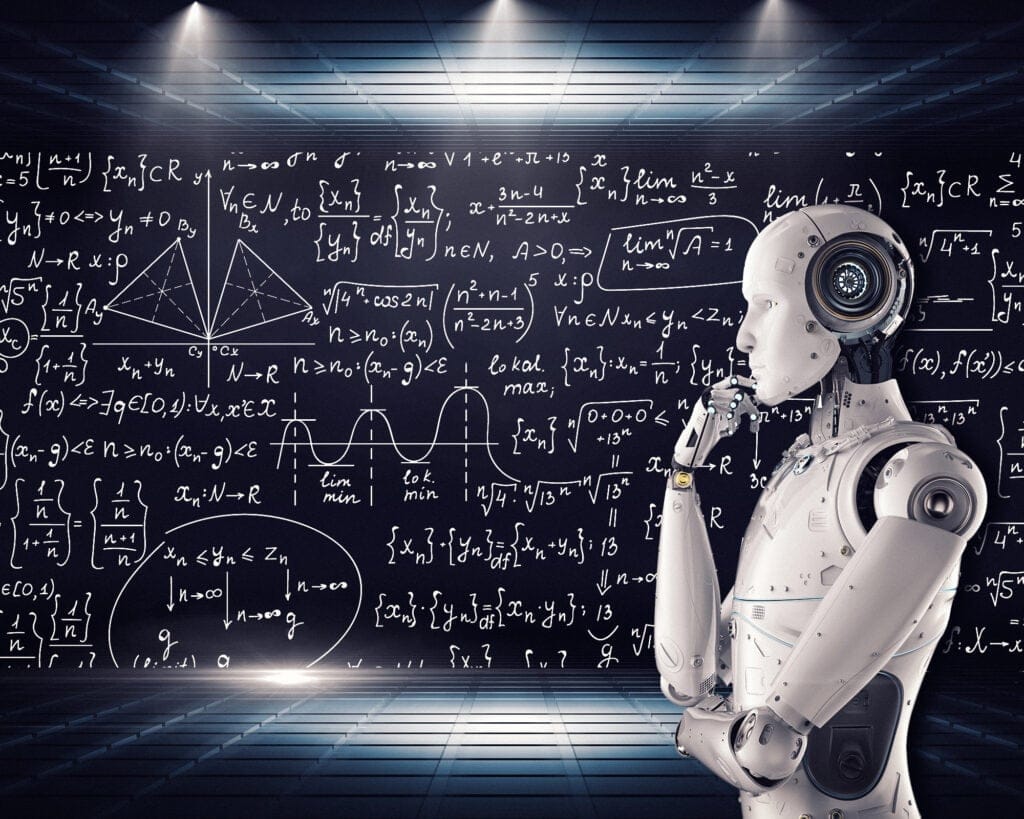
Introducing the Prompt Engineering Toolkit
Products
Company