Announcing a New Framework for Designing Optimal Experiments with Pyro
12 May 2020 / Global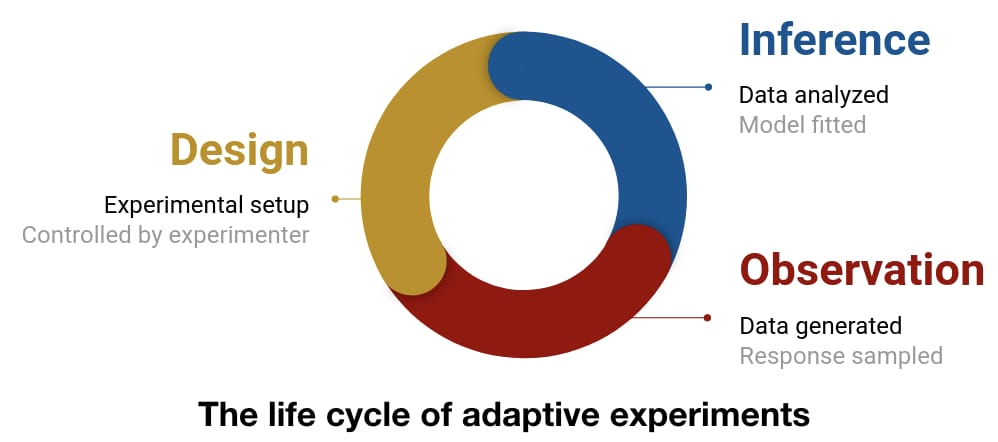
Experimentation is one of humanity’s principal tools for learning about our complex world. Advances in knowledge from medicine to psychology require a rigorous, iterative process in which we formulate hypotheses and test them by collecting and analyzing new evidence. At Uber, for example, experiments play an important role in the product development process, allowing us to roll out new variations that help us improve the user experience. Sometimes, we can rely on expert knowledge in designing these types of experiments, but for experiments with hundreds of design parameters, high-dimensional or noisy data, or where we need to adapt the design in real time, these insights alone won’t necessarily be up to the task.
To this end, AI researchers at Uber are looking into new methodologies for improving experimental design. One area of research we’ve recently explored makes use of optimal experimental design (OED), an established principle based on information theory that lets us automatically select designs for complex experiments.
In recent papers at NeurIPS and AISTATS written by researchers at Uber AI and the University of Oxford, we introduced a new class of algorithms for computing optimal experimental designs that is faster and more scalable than previous methods. We are also excited to provide a general purpose implementation of our OED framework built on top of Pyro, an open source probabilistic programming language created at Uber and hosted by the LF AI Foundation.
Our new framework lets experimenters automate OED on a giant class of models that can be expressed as Pyro programs. We hope that by open sourcing state-of-the-art experimental design algorithms, a wide range of experiments, from A/B tests to DNA assays, can be designed more easily.
The experimentation life cycle
Although every experiment is different, we can break down a typical experiment into three key stages:
-
- Design: Set up the controllable aspects of the experiment; this is where OED can be used.
- Observation: Conduct the experiment and obtain data.
- Inference: Analyze the data collected to update our model.
Importantly, this process usually continues after the first experiment. Once we have obtained some information about the phenomenon we are studying, we can use what we’ve learned to design the next experiment. It’s in this adaptive setting where OED can be particularly powerful. As we update our model, we adapt our experimental designs to ensure we obtain useful information from each experiment, allowing us to hone our knowledge efficiently.
Experimentation with Pyro
For a concrete example based on foundational psychology experiments done in the 1950s, suppose we want to learn the working memory capacity of a human participant in a psychology study. We’ll treat working memory capacity as the length of the longest list of random digits that the participant can memorize. Each step in our experiment proceeds as follows:
Design
We select the length of the list that we will ask the participant to try and remember. To do so, we score each possible design (using a scoring function that we define below) and pick the highest scoring design.
Observation
We present the participant with a list of random digits of the chosen length and record whether they remembered it correctly or not.
Inference
We use Bayesian inference to incorporate our new observation into an estimate of the participant’s working memory capacity. In this example, we will use a Bayesian logistic regression model. It models the probability of correctly remembering the list of digits of different lengths for people with different working memory capacities, as shown in Figure 1, below:
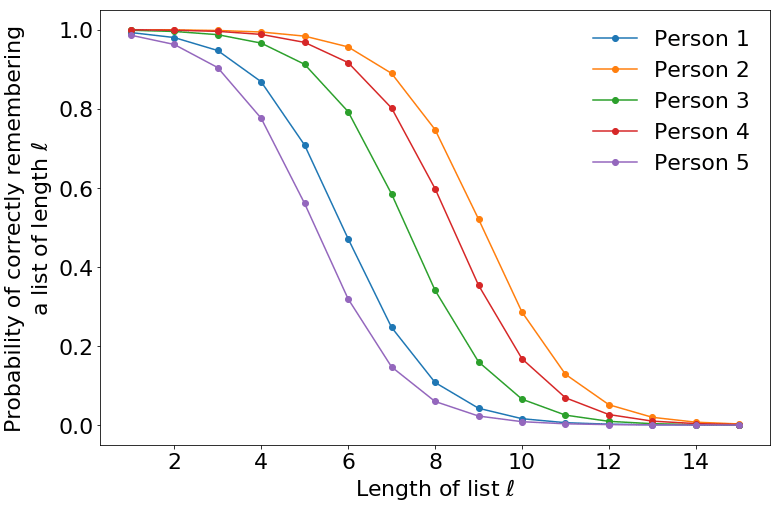
We also need a sense of what working memory capacities are plausible. Research suggests that across the general population, people can remember about seven digits with a standard deviation of two between different individuals.
Putting these modeling assumptions together, we can write a Pyro program that specifies our model for the memory capacity experiment:
(Note that here and throughout this article, we use somewhat simplified syntax so as not to distract from the big picture; for complete code snippets see the corresponding tutorial).
After each experiment, we leverage variational inference in Pyro to approximate our posterior distribution on the working memory capacity of the participant. Computing a posterior distribution at each iteration of the experiment is important because it allows us to make the optimal design choice based on all previously collected data.
Designing a sequential experiment
Now that we’ve defined our model, we can specify how the three stages of our experimental life cycle—design, observation, inference—interact in a series of experiments. Importantly, the outcomes of previous experiments are used to inform optimal design decisions in subsequent experiments.
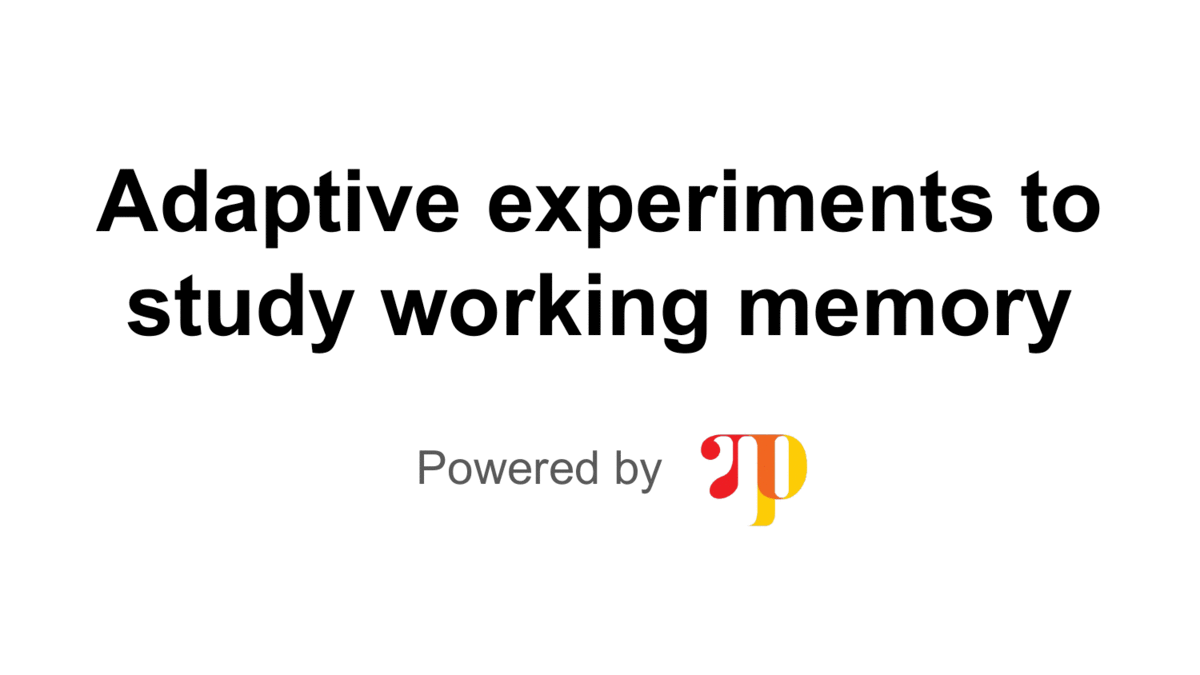
The following high-level code shows how a sequence of ten experiments can be planned and executed automatically:
Each loop of our experiment begins by scoring the possible designs using experiment_design_score and picking the best one. We haven’t yet said how this scoring function will work—we’ll pick that up in the next section of the article.
Next, our code gets a response from the user by showing them a list of random digits of the chosen length using human_response, a function that handles the UI aspects of the experiment. Finally, we update our beliefs by calling run_inference using all the data we have obtained so far.
For an implementation of experiment_design_score, we first need to define a quality score for designs and then find a way to efficiently estimate it.
What is the optimal experimental design?
There are a number of ways to score experimental designs; in our work we focus on the expected information gain (EIG), which is a measure of the information we expect to gain from doing an experiment. To understand what EIG is measuring, we first need to understand what constitutes a useful experiment. Let’s do this by studying two examples. In the first, we pick an experimental design, conduct the experiment, compute the posterior, and end up with a posterior distribution that is barely different from the prior, as shown in Figure 3, below:
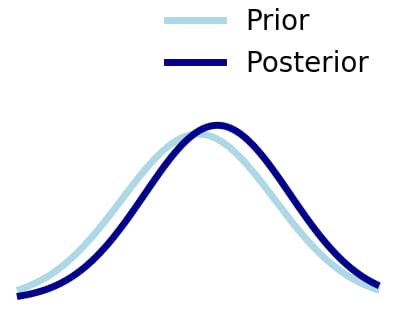
This experiment isn’t particularly useful because our level of uncertainty about the true value of the target is pretty much the same as it was initially. A better experiment would be one that leads to a posterior that is more peaked than the prior, as shown in Figure 4, below:
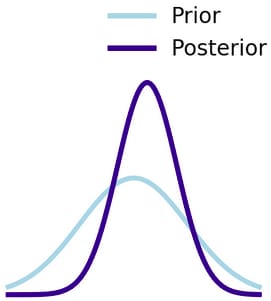
Here, the uncertainty has decreased as a result of the new data. This is a general feature of useful experiments.
This decrease can be made quantitative in terms of entropy, a measure of the uncertainty in a distribution that works well for both discrete and continuous variables. We use it to define the information gain of an experiment as:
Information gain = entropy of prior – entropy of posterior
This quantity measures how much we reduced uncertainty about the target, the unknown that we are trying to learn about, by doing the experiment. The problem with information gain as defined here is that we cannot calculate it until after the experiment has been conducted, since computing the posterior requires the actual observation. To circumvent this, we instead score experimental designs on expected information gain, which is the expectation of the information gain over all the possible observations we might end up with if we ran the experiment.
Intuitively, we simulate a large number of possible experimental outcomes given our current knowledge of the world. We then compute the information gain for each outcome. Aggregating all these individual information gains then yields the EIG.
The EIG depends on the prior to tell us what we already know and where we might hope to improve our knowledge. In adaptive experiments, the prior accounts for all the data that we have seen so far. This dependency on the prior allows the optimal experimental design to change as more knowledge is gained to identify where the most uncertainty still remains.
Computing the optimal design
Our score for experimental designs, EIG, is notoriously difficult to estimate. In our NeurIPS paper about our approach to OED, we proposed four variational methods for EIG estimation, each suited to different modelling assumptions. In this article, we introduce the simplest one, called the marginal method.
In order to define the marginal method, we first express the EIG in a different manner from that described above:
EIG = Total entropy of experiment outcome – Expected entropy of observation noise
In this equation, observation noise refers to the uncertainty that cannot be removed even when we know the exact value of the target.
For example, in the case of working memory, the total entropy of the outcome combines uncertainty about the participant’s true working memory with uncertainty from the fact that the participant can make a mistake on an easy list or get lucky on a difficult one. The expected entropy of the observation noise only considers the latter source of uncertainty.
Fortunately, the expected entropy of the observation noise is quite easy to estimate. To compute the total entropy of the experiment outcome we need to know the marginal distribution of the outcome, which is rarely available in practice. In our marginal method we make a variational density approximation to the marginal distribution. We proved that this leads to an upper bound on the EIG:
EIG ≤ Total entropy of variational approximation – Expected entropy of observation noise
We can minimize this upper bound by improving our variational approximation, which should lead to a good approximation to the EIG. In our paper, we showed that this method can be remarkably accurate on a range of different experimental design problems.
Finally, given a way of estimating the EIG, we need to search over possible designs to find the best one. If the number of designs is small this can be done with exhaustive search. For larger design spaces sensible strategies include Bayesian optimization (e.g., with BoTorch) or gradient ascent methods.
Let’s see the marginal method in practice in the working memory example.
Estimating EIG in Pyro
So far, we have an experimental loop set-up to run 10 experiments—we just need to fill in the experiment_design_score function to get the best design at each iteration. Here we will use the marginal method to approximate the EIG.
To form a variational approximation to the marginal distribution, we need a family of approximating distributions (in Pyro these are called ‘guides’). Since the outcome remembered_correct is a binary variable, the natural choice is a Bernoulli distribution:
To estimate the EIG, we will train the parameter q_logitto minimize the upper bound on EIG, and as such, provide accurate EIG estimates. Fortunately, Pyro takes care of most of this for us:
This code will run 1,000 steps of gradient descent on q_logit to tighten the upper bound on EIG, and then make a 104 sample estimate of the EIG using this approximation. Figure 5, below, shows the computed EIG scores for the very first round of experimentation, before any data has been collected:
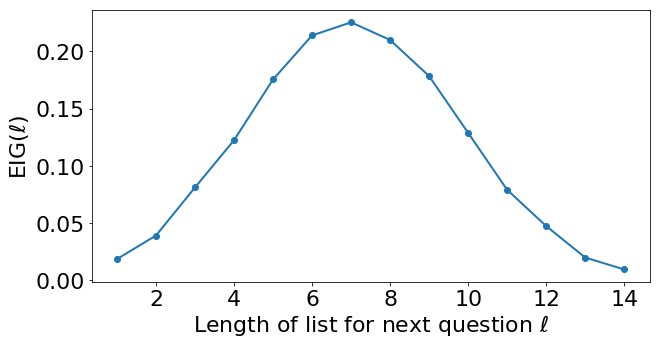
We can see that the best designs are near the prior mean of seven, where we expect some but not all participants to be able to remember the list of digits correctly. This makes intuitive sense; if we ask a participant to remember a very short list of digits, although they are almost certain to get it right, we’ll have learned very little about their working memory capacity.
Conversely, if we ask a participant to remember an extremely long list of digits, they will almost certainly memorize it incorrectly, and we’ll have learned very little about their working memory capacity. The sweet spot (in other words, the optimal design) is somewhere in the middle. The whole point of OED is to define this sweet spot in a quantitative way that can be automated.
Below, we demonstrate the output of running multiple steps of adaptive experiments with one participant:
We see, for example, that if the participant gets the first question wrong, our next experiment should use a list of digits of length 5.
Figure 6, below, shows how the posterior changes as we collect data from a sequential adaptive experiment:
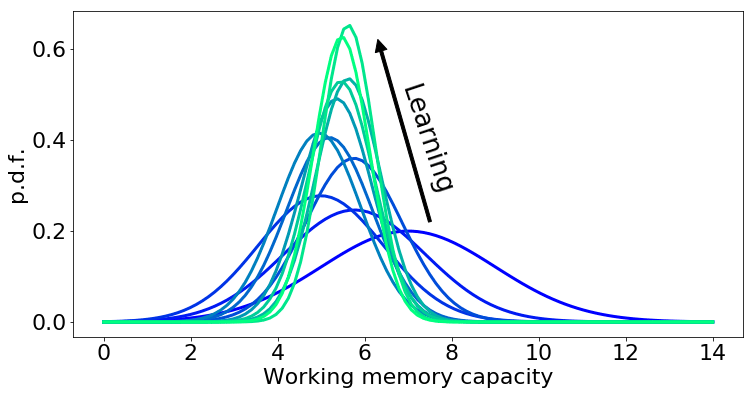
In this example, the prior is shown in blue with the posterior after each of 10 experiments depicted in various shades of blue/green. We can see that as we collect more data the posterior sharpens around a working memory capacity of 5.5.
Finally, let’s compare OED to a heuristic design strategy in which we run 10 experiments in which we ask the participant to memorize lists of length 1, 2, …, 10.
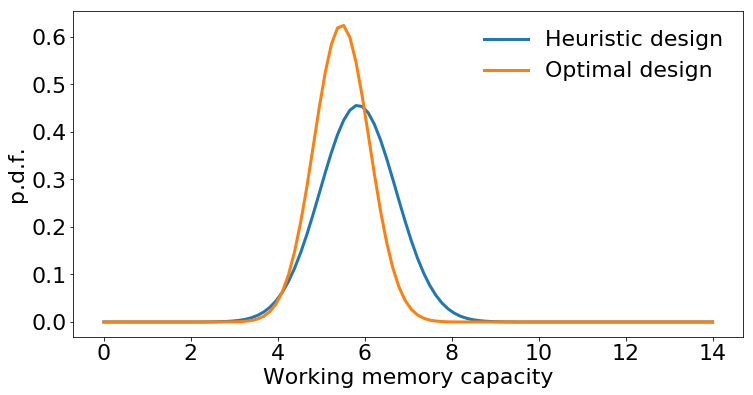
It’s clear that using optimal designs has led to a firmer conclusion after the same number of trials. In other words, OED has allowed us to learn more about the world using the same experimental budget. While this may not be a big deal for a simple experiment such as ours, it can be very difficult to find good design heuristics for more complex experiments—and almost impossible to find good heuristics that are adaptive. This is why OED is so attractive: it automates experimental design and makes experiments more efficient.
Designing other kinds of experiments
We’ve walked through how to design adaptive experiments using the marginal method above. This method is fairly flexible. For example, it could work equally well if the observation was continuous rather than discrete (although in that case we would need a different marginal_guide).
There are other kinds of models where the marginal method isn’t applicable. Specifically, in the working memory example there are no other variables aside from the target working_mem_capacity and remembered_correct. Sometimes there are additional nuisance variables in the model, and in this case the ‘Expected entropy of observation noise’ term in the EIG is no longer easy to calculate so the marginal method doesn’t work.
An alternative estimator, also from our NeurIPS paper, is the posterior estimator. As its name suggests, this estimator makes use of a variational approximation to the posterior rather than the marginal distribution and can be used in models with nuisance variables. In this Pyro tutorial, we show how to use the posterior estimator to find a polling strategy to make more accurate predictions of the winner of a U.S. presidential election (the target) without needing to get accurate estimates of the voting patterns in each state (the nuisance variables).
Another potential limitation is the size of the design space. The approach taken in the working memory example was to score every possible design and then pick the best one. Unsurprisingly, this doesn’t scale to large design spaces. In our AISTATS paper, however, we showed that for continuous designs, we can learn the design and improve our variational approximation simultaneously using gradient ascent. This is attractive because it means we can locate the optimal design without having to visit most of the design space.
Looking forward
Tools for designing experiments optimally and automatically are of immense value in a wide range of applications. For example, neuroscientists use OED to map neural microcircuits in the brain by adaptively choosing which neurons to stimulate; psychologists compare competing models of human memory using trials that were adapted to become more difficult for participants with better memories; and OED is used in clinical trial design to choose optimal sample sizes.
By developing and open sourcing OED in Pyro, we hope others in the community can benefit from this framework and apply it to their own research areas. To learn more about variational algorithms for Bayesian optimal experimental design, check out our recent papers that introduce variational OED and extend it to high-dimensional designs.
For more details on OED in Pyro, including code, see the tutorial based on the working memory example covered above as well as another on designing electoral polls.
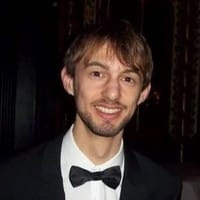
Adam Foster
Adam Foster was a research intern at Uber and is currently pursuing a Ph.D. at the University of Oxford.
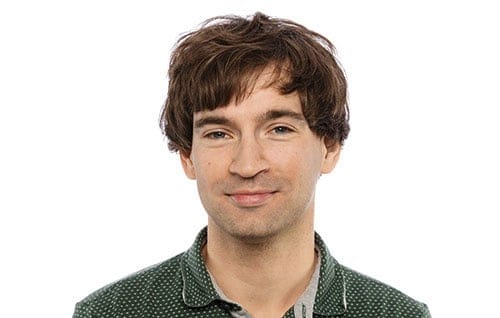
Martin Jankowiak
Martin Jankowiak is a senior research scientist at Uber whose research focuses on probabilistic machine learning. He is a co-creator of the Pyro probabilistic programming language.
Posted by Adam Foster, Martin Jankowiak
Related articles
Most popular
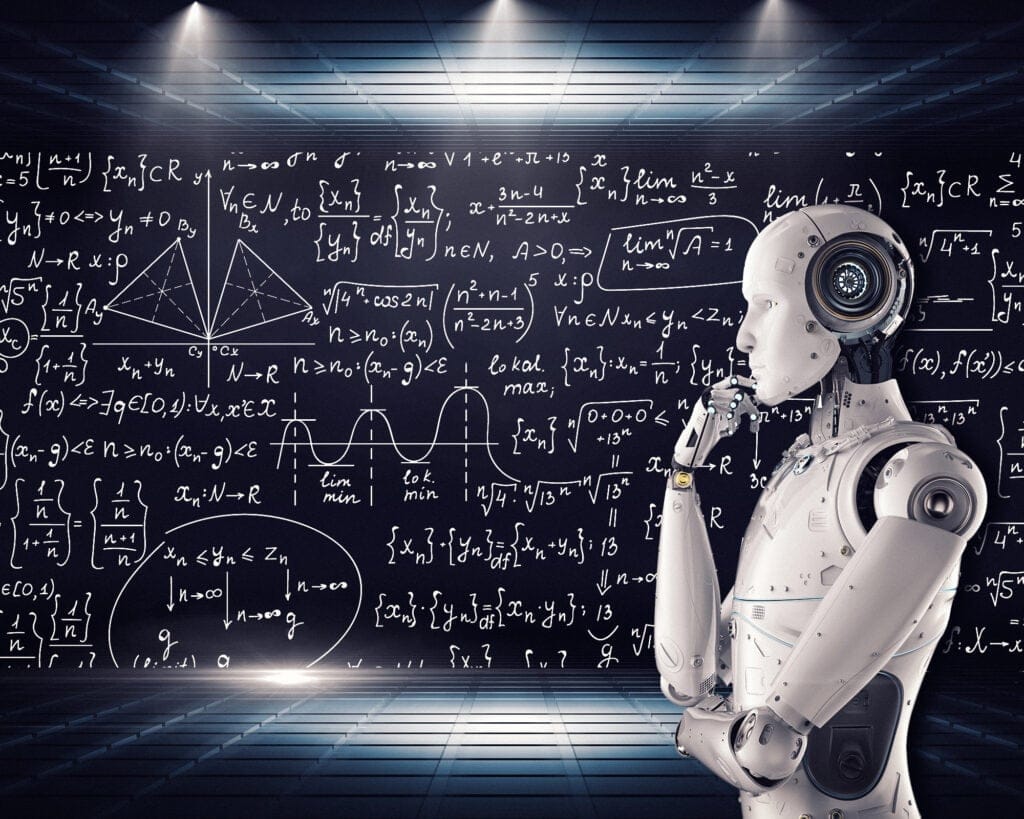
Introducing the Prompt Engineering Toolkit
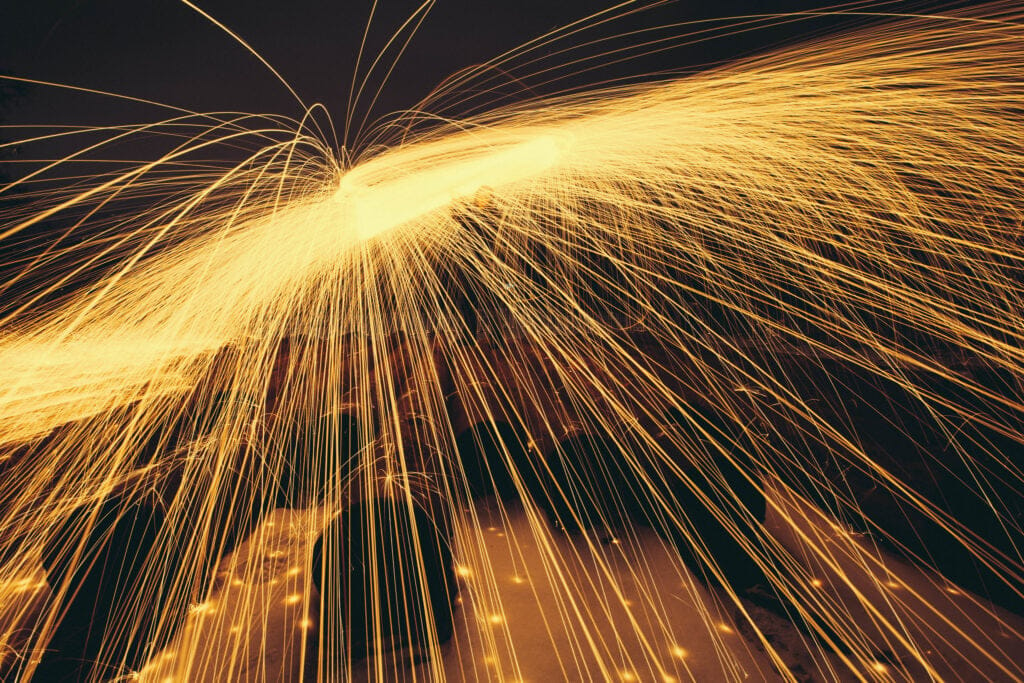
Serving Millions of Apache Pinot™ Queries with Neutrino
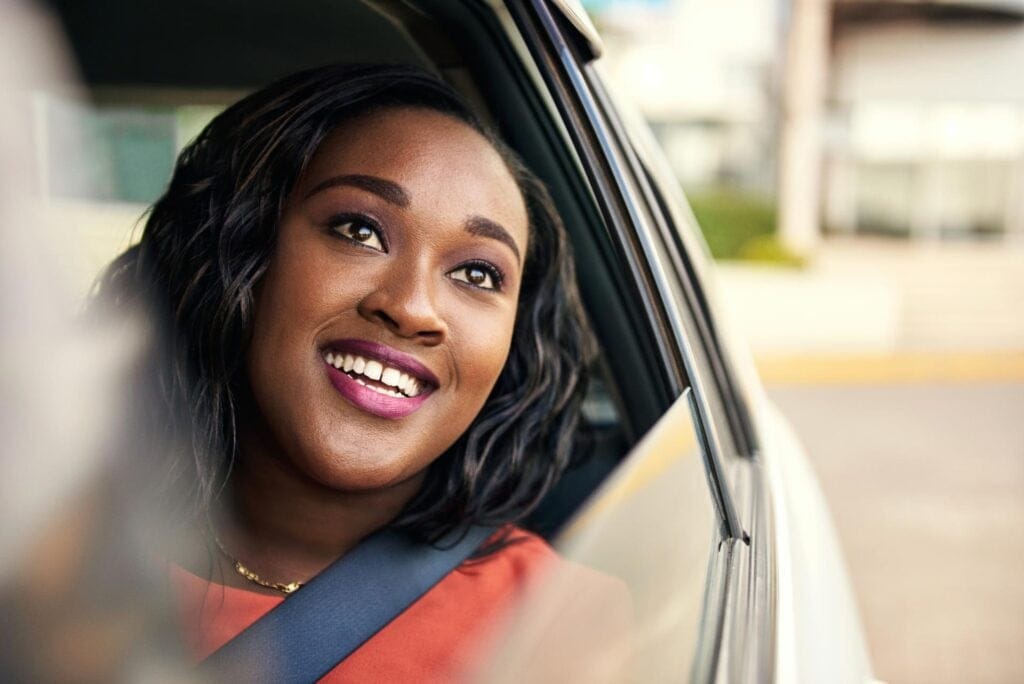
Your guide to NJ TRANSIT’s Access Link Riders’ Choice Pilot 2.0
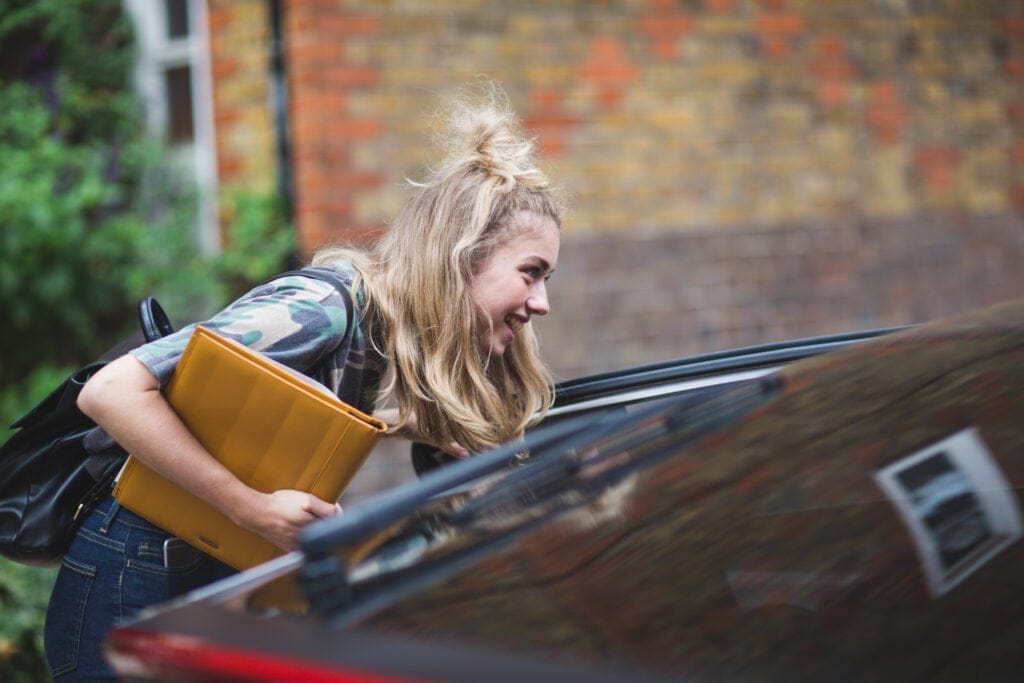
Connecting communities: how Harrisburg University expands transportation access with Uber
Products
Company