Introducing Petastorm: Uber ATG’s Data Access Library for Deep Learning
September 21, 2018 / Global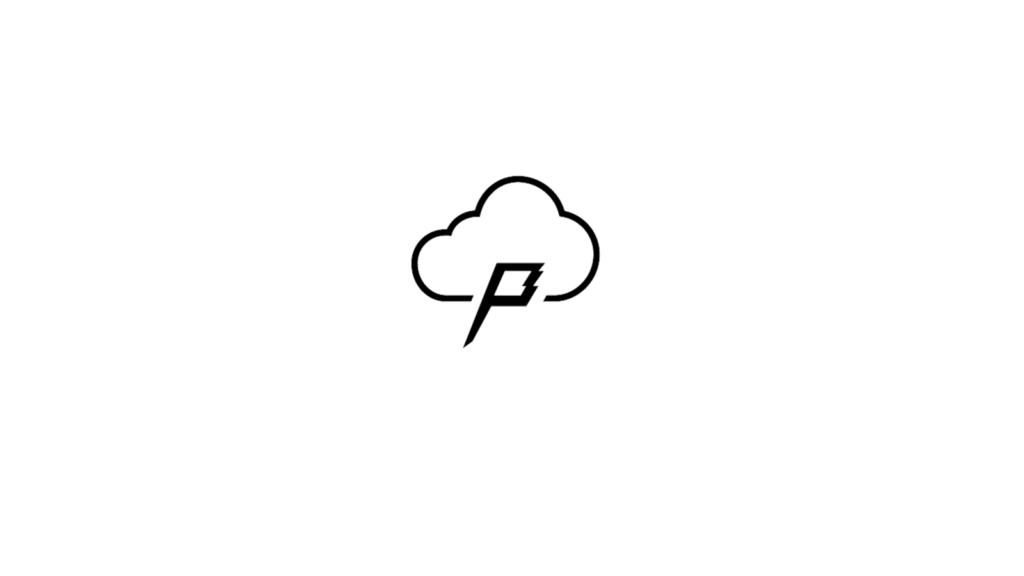
In recent years, deep learning has taken a central role in solving a wide range of problems in pattern recognition. At Uber Advanced Technologies Group (ATG), we use deep learning to solve various problems in the autonomous driving space, since many of these are pattern recognition problems. Many of our models require tens of terabytes of training data acquired from numerous sensors, including cameras, lidars, and radars.
Researchers and engineers at Uber ATG are actively pushing the state of the art in autonomous driving across multiple problem domains, such as perception, prediction and planning. To support these efforts, our team is working on developing dataset storage solutions that will make data more easily available to researchers, allowing them to focus on model experimentation.
In this article, we describe Petastorm, an open source data access library developed at Uber ATG. This library enables single machine or distributed training and evaluation of deep learning models directly from multi-terabyte datasets in Apache Parquet format. Petastorm supports popular Python-based machine learning (ML) frameworks such as Tensorflow, Pytorch, and PySpark. It can also be used from pure Python code.
A deep learning cluster setup
Training state-of-the art models takes time even on modern hardware, and in many cases, distributing the training load on multiple machines is essential. A typical deep learning cluster performs the following steps:
- One or more machines read samples from a centralized or a local dataset.
- Individual machines evaluate the value of a loss function and compute its gradient with respect to model parameters. GPU cards are typically used during this step.
- Model coefficients are updated by combining estimated gradients, often calculated by multiple machines in a distributed fashion (e.g., using our open source Horovod library, a deep learning framework).
One such setup is shown in Figure 1, below:
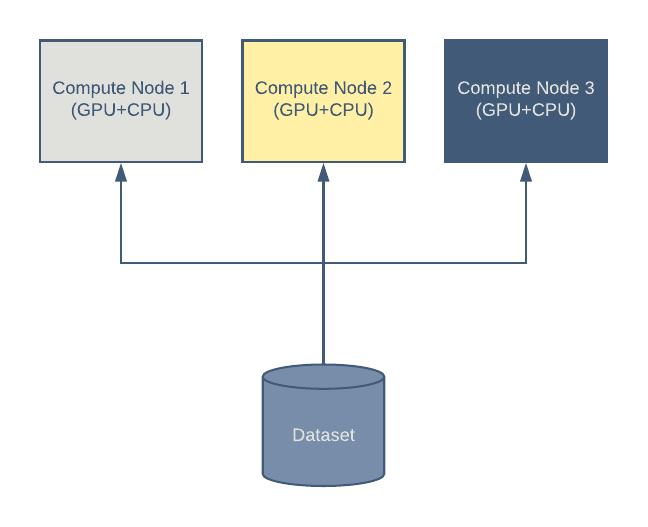
Given the expense of GPUs, good performance utilization of the GPU-enabled cluster is essential. A well-tuned data access layer ensures that the data used for training is always available to the GPUs so that the GPUs are never idle. In this setup, the data flows directly from the storage to individual nodes.
Streamlining model architecture research
Preparing a multi-terabyte dataset that contains properly synchronized data from a multiplicity of data sources is often an error-prone task. We want to give researchers a single dataset that allows them to work on a wide range of tasks, eliminating the need to create a new dataset for each problem.
For this to happen, the following principles need to be observed:
- Datasets contain a superset of the data a researcher might need, so they can pick and choose subsets of columns and rows for a particular experiment. Making this work at scale requires a careful choice of technology.
- Sensor data stored in the dataset is minimally pre-processed. We encourage researchers to implement all preprocessing on-the-fly as a part of training/evaluation procedures. In many cases, this is possible by using otherwise underutilized CPUs.
Common industry patterns for dataset storage in deep learning applications typically fall into two categories: multi-file and record streaming datasets. In the following sections, we describe them in more detail.
Multifile datasets
In this case, each tensor/image/set-of-labels is stored in a separate file (e.g., PNG, JPEG, NPZ, and CSV). The entire dataset is stored as one or more file system directories, with each containing numerous files. The number of files may reach millions (for instance, ImageNet has 1.2 million files). Datasets used by Uber ATG would have more than 100 million files if stored in this format.
This approach gives users random access to any column of any row in the dataset. However, multiple round-trips to the filesystem are costly. It is hard to implement at large scale, especially using modern distributed file systems such as HDFS and S3 (these systems are typically optimized for fast reads of large chunks of data).
Record streaming datasets
Alternatively, sets of rows are grouped together into one or more files. For example, Tensorflow uses a protobuf file (TFRecord). Other popular formats include HDF5 and Python pickle files.
This approach works well with the HDFS and S3 file systems. However, querying a particular column requires transmitting all fields over the wire and then discarding unused data. Querying for a single row also demands custom index implementation.
After evaluating multiple options, we decided to leverage the Apache Parquet storage format, which mitigates some of the shortcomings of these two approaches:
- Facilitates large continuous reads (HDFS/S3-friendly)
- Supports fast access to individual columns
- Allows faster row queries in some cases
- Integrates well with Apache Spark, as an off-the-shelf query/manipulation framework
Columnar stores and Apache Parquet
Columnar data stores organize table data in a column-wise rather than row-wise order. For example, a table of data recorded off an autonomous vehicle sensor may look like this:
Row | camera #1 | camera #2 | Lidar | Labels |
1 | <camera1-1> | <camera2-1> | <lidar 1> | <labels 1> |
2 | <camera1-2> | <camera2-2> | <lidar 2> | <labels 2> |
3 | <camera1-3> | <camera2-3> | <lidar 3> | <labels 3> |
The differences between row and columnar storage are shown below:
Row storage | Columnar storage | ||
row 1 | <camera1-1> | <camera1-1> | |
<camera2-1> | <camera1-2> | ||
<lidar 1> | <camera1-3> | ||
<labels 1> | <camera2-1> | ||
row 2 | <camera1-2> | <camera2-2> | |
<camera2-2> | <camera2-3> | ||
<lidar 2> | <lidar 1> | ||
<labels 2> | <lidar 2> | ||
row 3 | <camera1-3> | <lidar 3> | |
<camera2-3> | <labels 1> | ||
<lidar 3> | <labels 2> | ||
<labels 3> | <labels 3> |
Storing data in a columnar order allows a user to load only a subset of columns, hence reducing the amount of data transmitted over the wire. In the case of rich sensor data from a self-driving vehicle, the benefit could be significant: consider loading just one image out of 10 high resolution images stored in the same row if your experiment only uses images from that single camera.
Apache Parquet is a columnar storage format that has become popular in recent years. It is well-supported by Apache Spark, Apache Arrow, and other open source projects, and it possesses the properties required for streamlining model architecture research.
Tensorflow and Pytorch are frameworks commonly used by the deep learning community. These frameworks do not natively support Parquet storage access, so we built Petastorm to bridge that gap.
Introducing Petastorm
Typically, a dataset is generated by joining records from several data sources. This dataset, generated by Apache Spark’s Python interface, PySpark, is later consumed by an ML training procedure. Petastorm provides a simple function that augments a standard Parquet store with a Petastorm specific metadata, thereby making it compatible with Petastorm.
With Petastorm, consuming data is as simple as creating a reader object from an HDFS or filesystem path and iterating over it. Petastorm uses the PyArrow library to read Parquet files. The high-level overview of this process is shown in Figure 2, below:
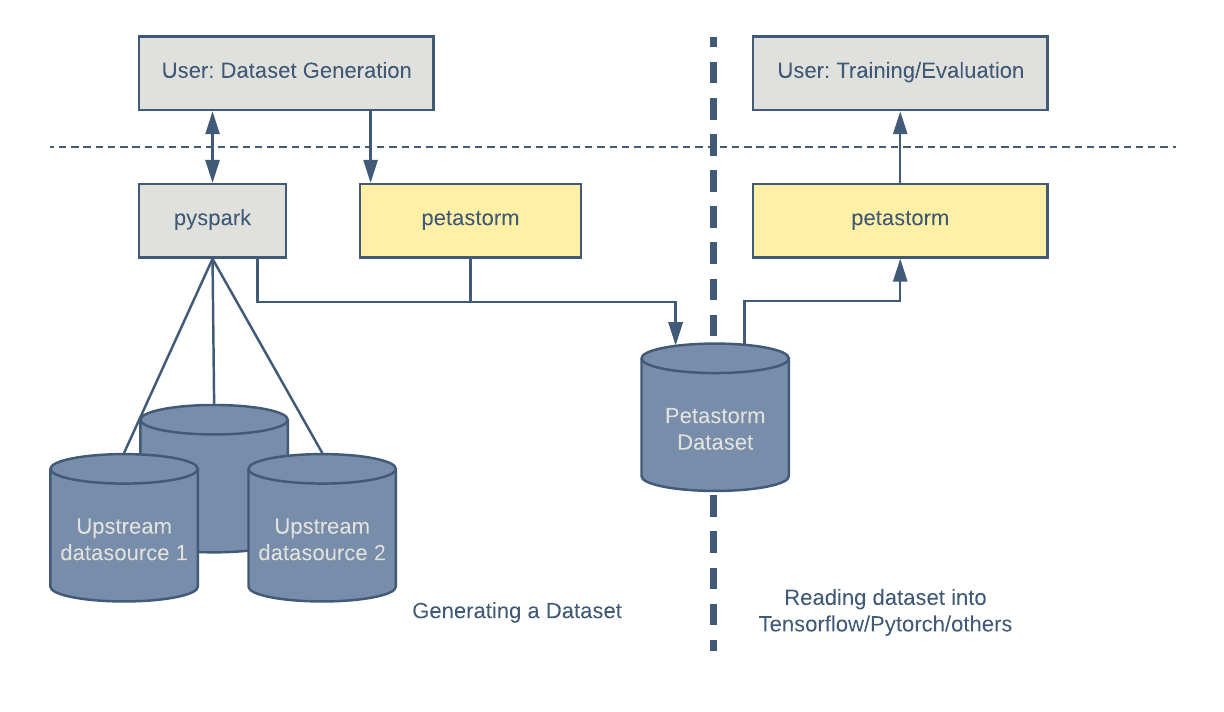
Generating a dataset
To generate a dataset with Petastorm, a user first needs to define a data schema, referred to as a Unischema. This is the only time a user needs to define a schema since Petastorm translates it into all supported framework formats, such as PySpark, Tensorflow, and pure Python.
An instance of Unischema is serialized as a custom field into a Parquet store metadata, hence a path to a dataset is sufficient for reading it.
The following example shows how a Unischema instance is created. Required field properties include: field name, a data type (represented by a NumPy data type), multidimensional array shape, a codec used for data encoding/decoding, and whether a field is nullable or not.
HelloWorldSchema = Unischema('HelloWorldSchema', [
UnischemaField('id', np.int32, (), ScalarCodec(IntegerType()), False),
UnischemaField('image1', np.uint8, (128, 256, 3) CompressedImageCodec('png'), False),
UnischemaField('array_4d', np.uint8, (None, 128, 30, None), NdarrayCodec(), False),
])
We use PySpark to write the Petastorm dataset. The following example shows how a 1,000 row dataset is created using our library.
rows_count = 10
with materialize_dataset(spark, output_url, HelloWorldSchema, rowgroup_size_mb):
rows_rdd = sc.parallelize(range(rows_count))
.map(row_generator)
.map(lambda x: dict_to_spark_row(HelloWorldSchema, x))
spark.createDataFrame(rows_rdd, HelloWorldSchema.as_spark_schema())
.write
.parquet('file:///tmp/hello_world_dataset')
- materialize_dataset context manager performs necessary configurations at the beginning and writes out Petastorm-specific metadata at the end. The output URL could point either to an HDFS or filesystem location.
- Rowgroup_size_mb defines the target size of Parquet row group in megabytes.
- row_generator is a function that returns a Python dictionary matching the HelloWorldSchema.
- dict_to_spark_row validates data types according to the HelloWorldSchema and converts the dictionary into a pyspark.Row object.
Reading a Dataset
Next, we outline how to read a dataset from plain Python code, as well as from two commonly used machine learning frameworks: Tensorflow and Pytorch.
Python
A Reader instance can access a Petastorm dataset directly from Python code. Reader implements the iterator interface, hence going over the samples is very straightforward:
with Reader('file:///tmp/hello_world_dataset') as reader:
# Pure python
for sample in reader:
print(sample.id)
plt.imshow(sample.image1)
Tensorflow
The following example shows how to stream a dataset into Tensorflow. examples is a named tuple with the keys automatically derived from the Unischema, and the values are tf.tensor objects:
with Reader('file:///tmp/hello_world_dataset') as reader:
tensor = tf_tensors(reader)
with tf.Session() as sess:
sample = sess.run(tensor)
print(sample.id)
plt.imshow(sample.image1)
In the near future, a user will be able to access the data using the tf.data.Dataset interface.
Pytorch
A Petastorm dataset can be incorporated into Pytorch via an adapter class, petastorm.pytorch.DataLoader, as follows:
with DataLoader(Reader('file:///tmp/hello_world_dataset')) as train_loader:
sample = next(iter(train_loader))
print(sample['id'])
plt.plot(sample['image1'])
Analyzing a dataset using Spark
Using the Parquet data format, which is natively supported by Spark, makes it possible to use a wide range of Spark tools to analyze and manipulate the dataset. The example below shows how to read a Petastorm dataset as a Spark RDD object:
rdd = dataset_as_rdd('file:///tmp/hello_world_dataset', spark,
[HelloWorldSchema.id, HelloWorldSchema.image1])
print(rdd.first().id)
Standard PySpark tools can be used to work with the Petastorm dataset. Note that the data is not decoded and only values of the fields that have a corresponding native representation in Parquet format (e.g. scalars) are meaningful:
# Create a dataframe object from a parquet file
dataframe = spark.read.parquet(dataset_url)
# Show a schema
dataframe.printSchema()
# Count all
dataframe.count()
# Show a single column
dataframe.select('id').show()
SQL can be used to query a Petastorm dataset:
number_of_rows = spark.sql(
'SELECT count(id) '
'from parquet.`file:///tmp/hello_world_dataset`').collect()
Petastorm features
Petastorm incorporates various features to support training scenarios for autonomous driving algorithms. These include efficient implementations of row filtering, data sharding, shuffling, access to subset of fields as well as support of time-series data (n-grams).
For additional context, the structure of a typical dataset include:
- Multiple columns with sensor-acquired signals collected during autonomous vehicle test runs. These include cameras, lidar, and radars.
- Manually generated labels stored as fields in a row.
Rows are sorted in a chronological order grouped by runs. Row group size is typically within the range of 30 to 100.
Parallel execution strategies
Petastorm offers two strategies for parallelizing data loading and decoding operations: one is based on a thread pool and another on a process pool implementation. Strategy choice depends on the kind of data being read.
Typically, the thread pool strategy should be used when a row contains encoded, high resolution images. In that case, most of the processing time is being used to decode the images via C++ code. No Python Global Interpreter Lock (GIL) is being held at that time.
Process pool strategy is more appropriate when row sizes are small. In this situation, most of the processing is done by pure Python code. More than one process must run in parallel in order to overcome execution serialization caused by GIL.
n-grams
Temporal context is needed for the models that can use dynamics of the observed environment in order to better interpret the environment and/or predict future behaviors of actors in the environment.
Petastorm can provide such temporal context when the underlying data is arranged by time. If an n-gram is requested from the Petastorm Reader object, consequent rows will be grouped into a single training sample.
The following diаgram shows the grouping for the n-gram of length 3. AV Log #0 and AV Log #1 show two distinct on-vehicle recordings of sensor data:
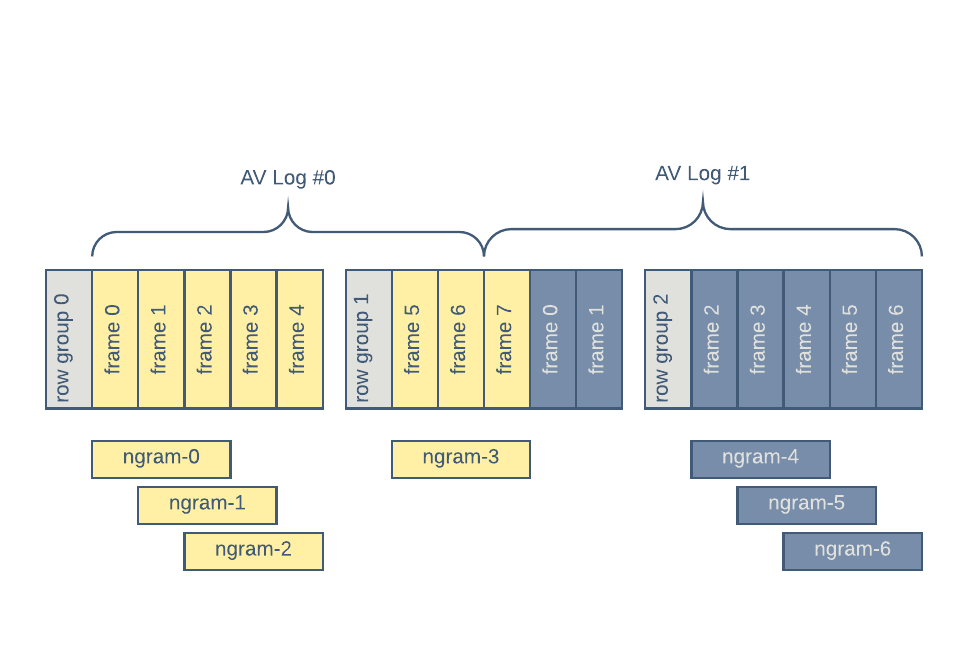
Note that groups of n-grams cannot span the Parquet row groups. In Figure 3, above, three 3-grams are generated from row-group 0; only one from row-group 1, and another three from row-group 2. n-grams save both IO and CPU bandwidth since there is no on-disk duplication of data nor duplicate loading/decoding.
n-grams are produced in the order they occur in the dataset so users need to make the order in the dataset match their access pattern.
Shuffling
If a dataset supports the n-gram access pattern, its rows are sorted by a timestamp. Parquet supports loading only the full amount of rows from a rowgroup. As a result, the data will be loaded in groups of highly correlated samples (e.g., two consequent camera images acquired from an autonomous-vehicle camera will be very similar). High correlation between consequent examples is undesirable and is known to reduce performance of training algorithms. To reduce correlation, Petastorm features shuffling.
Figure 4, below, illustrates different shuffling mechanisms built into Petastorm:
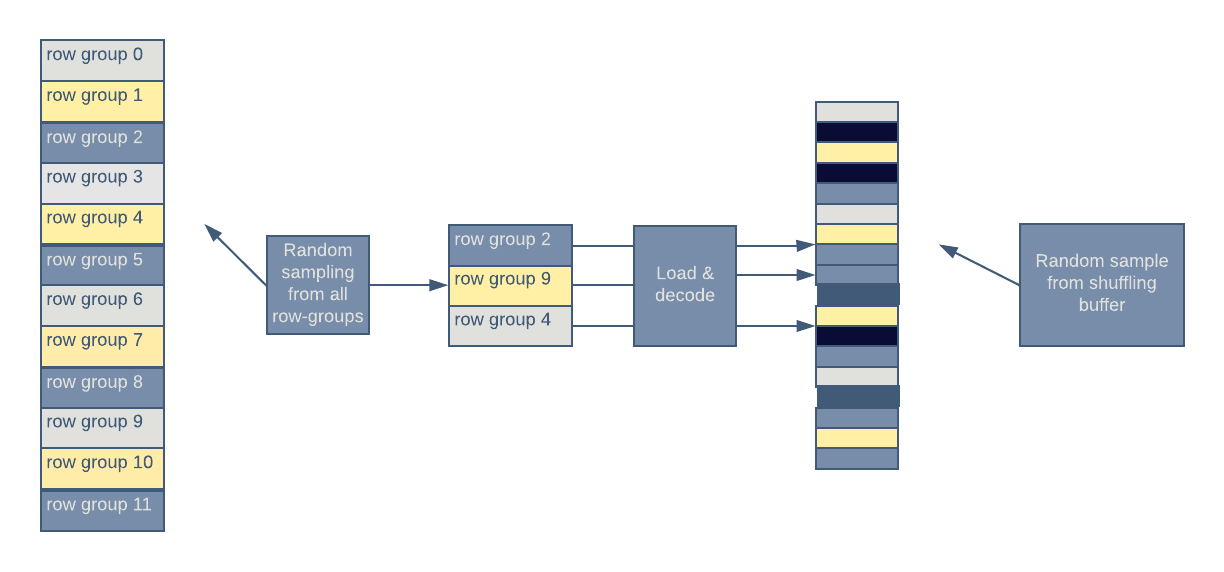
Petastorm randomly selects a set of row-groups from all row-groups in a dataset. Decoded rows are placed into the row shuffling buffer. A random row is selected from that buffer and returned to the user.
Row predicates (filters)
For a dataset instance to be reusable by multiple researchers in multiple experiments, it is important to be able to provide efficient mechanisms to select a subset of rows. Petastorm supports row predicates. Petastorm row predicates use Parquet store partitioning if available, and only the columns that are being conditioned upon are loaded from storage.
In the future, we plan to integrate support of Parquet’s predicate pushdown feature into Petastorm to further speedup queries.
Row group indexes
Petastorm supports storing a mapping between a key and a set of one or more row-groups together with a dataset. This mapping facilitates a quick lookup of row-groups that match specific criteria. Additional filtering is needed at a row level for which ‘row predicates’ may be used.
Sharding for distributed training
In a distributed training setup, each worker typically trains on a subset of data. That subset is orthogonal to the subsets provided to other machines participating in the training. Petastorm supports read-time sharding of a dataset into an orthogonal set of samples. Figure 5, below, demonstrates how Petastorm facilitates distributed training on a shareded dataset:
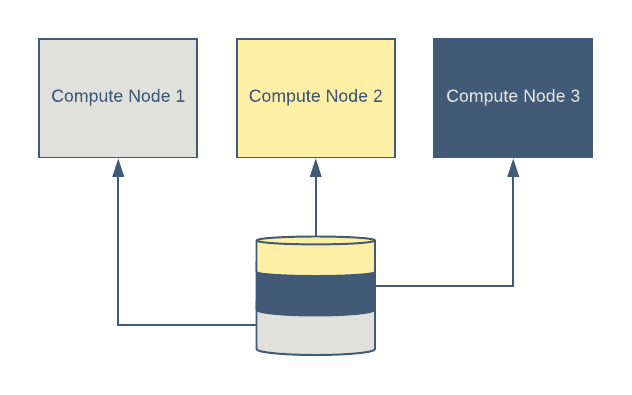
Local caching
Petastorm supports caching the data on local storage. This comes in handy when a network connection is slow or bandwidth is expensive. Figure 6, below, depicts this setup:
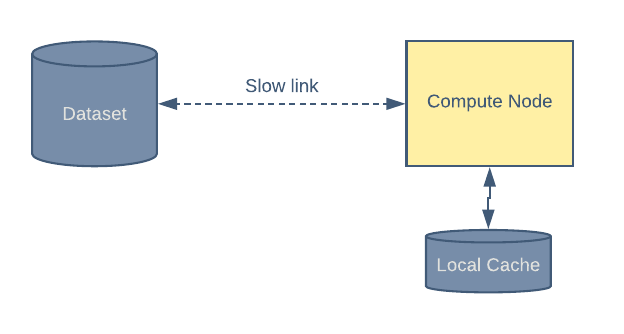
During the first epoch, a group of samples is read from the remote store and also saved into a local cache. During subsequent epochs, all data will be read from the local cache.
Petastorm architecture
Petastorm design goals include:
- Single data schema definition driving both encoding and decoding of data.
- High data loading bandwidth available to ML frameworks and pure Python code.
- Leveraging Apache Spark as a distributed cluster-compute framework for generating datasets.
- Pure Python, ML platform-agnostic implementation of core Petastorm components.
- Native look and feel of the interface presented to Tensorflow and PyTorch frameworks.
Figure 7, below, shows Petastorm components used during dataset generation and reading.
- etl package implements functionality for generating datasets.
- The Reader is the main data loading engine used by training/evaluation code. The Reader is implemented in pure Python and does not depend on any ML framework (Tensorflow, Pytorch), and can be instantiated by and used from plain Python code.
- Petastorm includes Tensorflow and PyTorch adaptors that present native interfaces for their respective frameworks.
- Unischema is referenced both by dataset generation and data loading code.
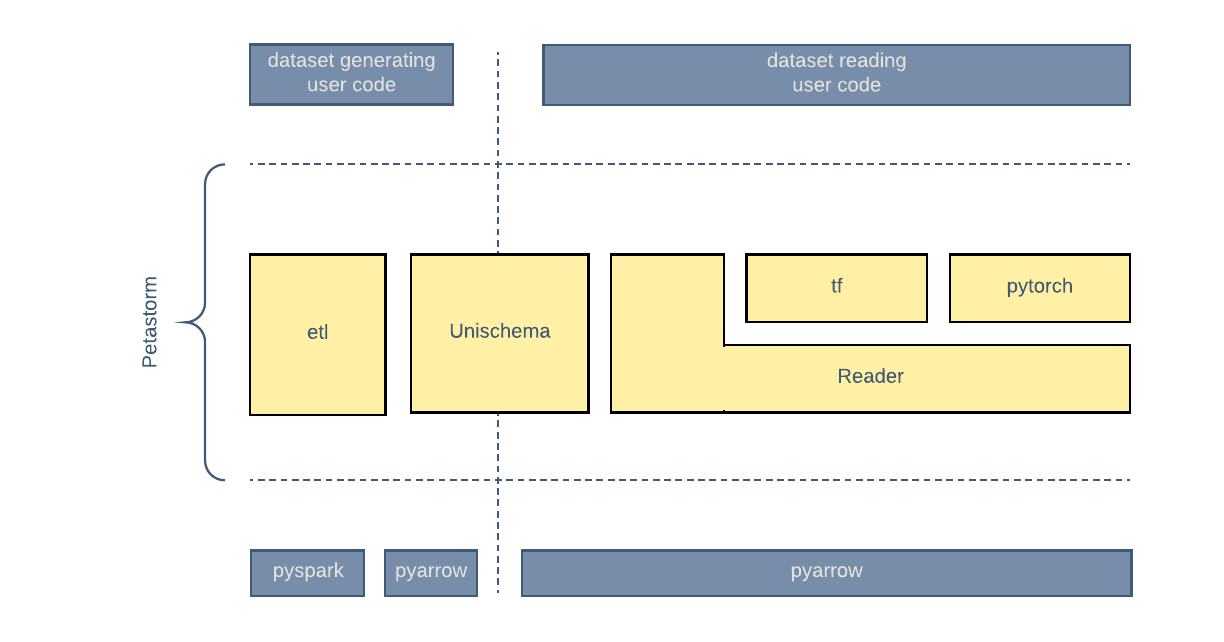
Parquet fixes
As we started working with Apache Parquet, we had some trouble getting Spark to write Parquet datasets with our data. The reason mainly came down to the size of our rows, which had several multi-megabyte fields. Our first problem was that row groups in our dataset were much larger than expected, causing issues such as out-of-memory. Digging into the code, we found that parquet-mr enforces an arbitrary minimum of 100 rows in a row group before checking if the row group has reached its target size set by the user. Assessing the project, there was already an issue filed along with a pull request, so we were able to fork the repo and port in the changes we needed to get more reasonable row group sizes.
Although we could achieve more reasonable row group sizes, we noticed that our Spark jobs would run out of memory when we tried to make smaller row groups or use even larger fields. By digging into the resulting datasets, the Parquet footer storing the file metadata was increasing significantly as we added new fields or reduced row group size.
It turned out that Parquet was generating statistics for our huge binary fields representing images or other multi-dimensional arrays. Since the Parquet statistics would store both a minimum and maximum value of each row group for these fields in the footer, the footer would grow too large to fit in memory if the row group size had decreased enough. Looking at the parquet-mr repository, this problem was already fixed; however, we were using Spark 2.1.0, which depends on Parquet 1.8.1. To address this, we upgraded our Spark distribution to use Parquet 1.8.3 (with the minimum row group size fix) and took advantage of these improvements.
Next steps
Below we highlight some of the improvements we plan to incorporate into Petastorm in the near future:
Reduce RAM footprint of random shuffling
Large row-groups help improve IO utilization and data loading rates. However, they also increase correlation between subsequent samples fed into a training algorithm. We are actively working on improved shuffling mechanisms.
Predicate pushdown support
Pyarrow will soon have predicate pushdown support. We would like to utilize it for faster row filtering.
Improved Spark integration
When accessing Petastorm datasets from Spark, some operations seem to take much more time or memory than expected. Further investigation into Parquet library code is needed to understand the additional nuances of efficiently working with very large fields.
Alternative storage formats
Petastorm abstracts the underlying storage format. Storage formats other than Parquet can be integrated into Petastorm, providing further freedom of experimentation and data loading performance tuning.
We hope Petastorm will help others create reusable, easy to use, and performant datasets. By open sourcing our work we hope to hear input and suggestions from the deep learning community. We welcome feedback and contributions: please report any issues you encounter, share speed-ups, and send pull requests.
If you are interested in working with Uber ATG to create machine learning systems for autonomous vehicles, consider applying for a role on our team!
Subscribe to our newsletter to keep up with the latest innovations from Uber Engineering.
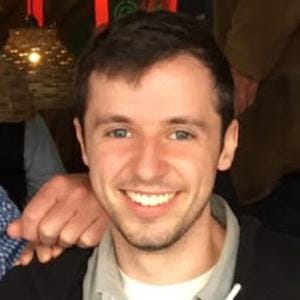
Robbie Gruener
Robbie Gruener is a software engineer on the Uber ATG Perception team.
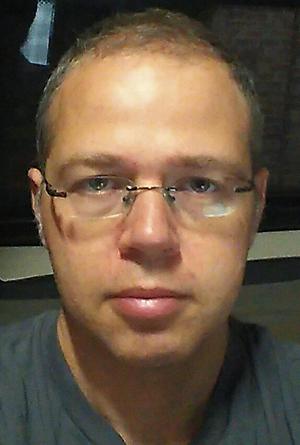
Yevgeni Litvin
Yevgeni Litvin is a senior software engineer on the Uber ATG Perception team.
Posted by Robbie Gruener, Owen Cheng, Yevgeni Litvin
Related articles
Most popular
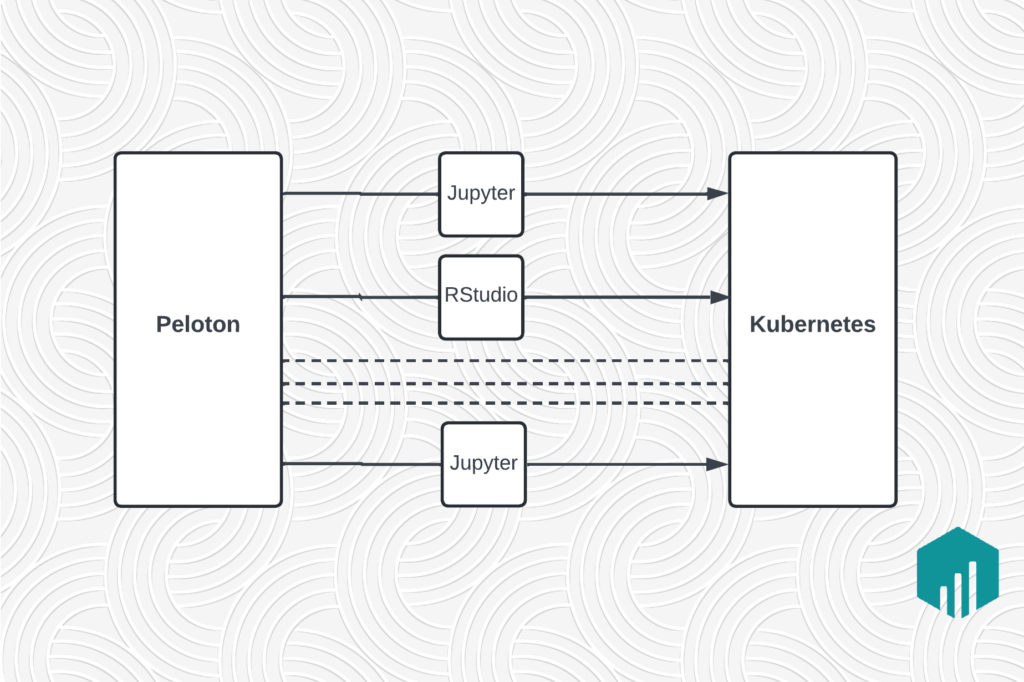
Migrating Large-Scale Interactive Compute Workloads to Kubernetes Without Disruption
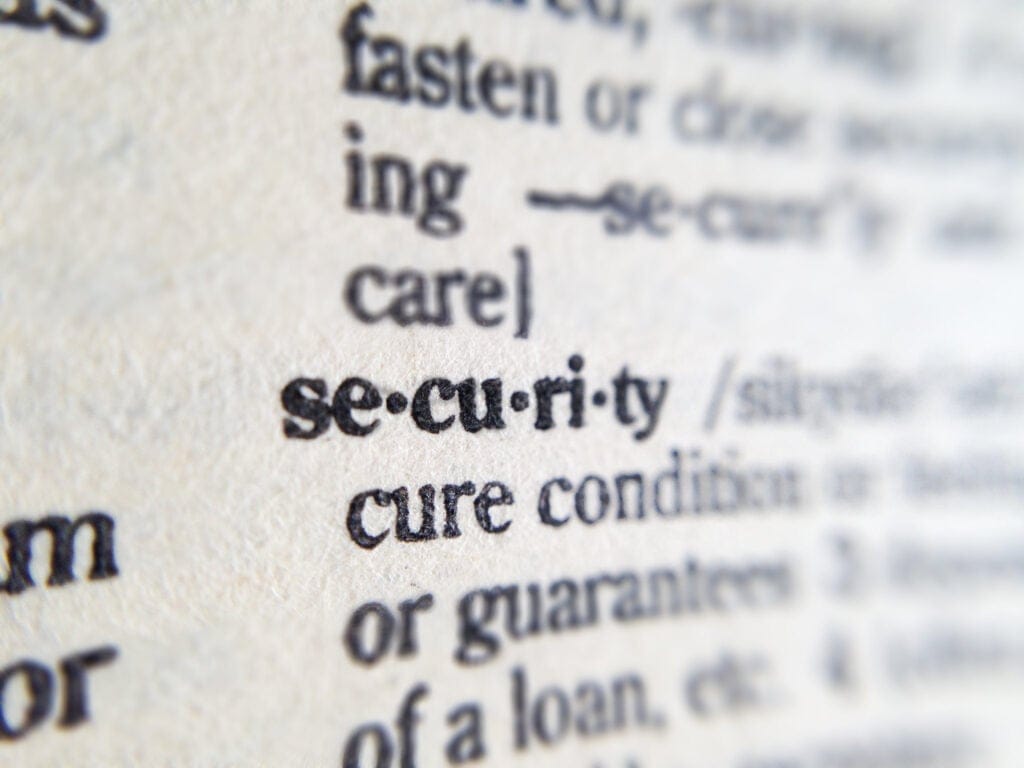
Building Uber’s Multi-Cloud Secrets Management Platform to Enhance Security
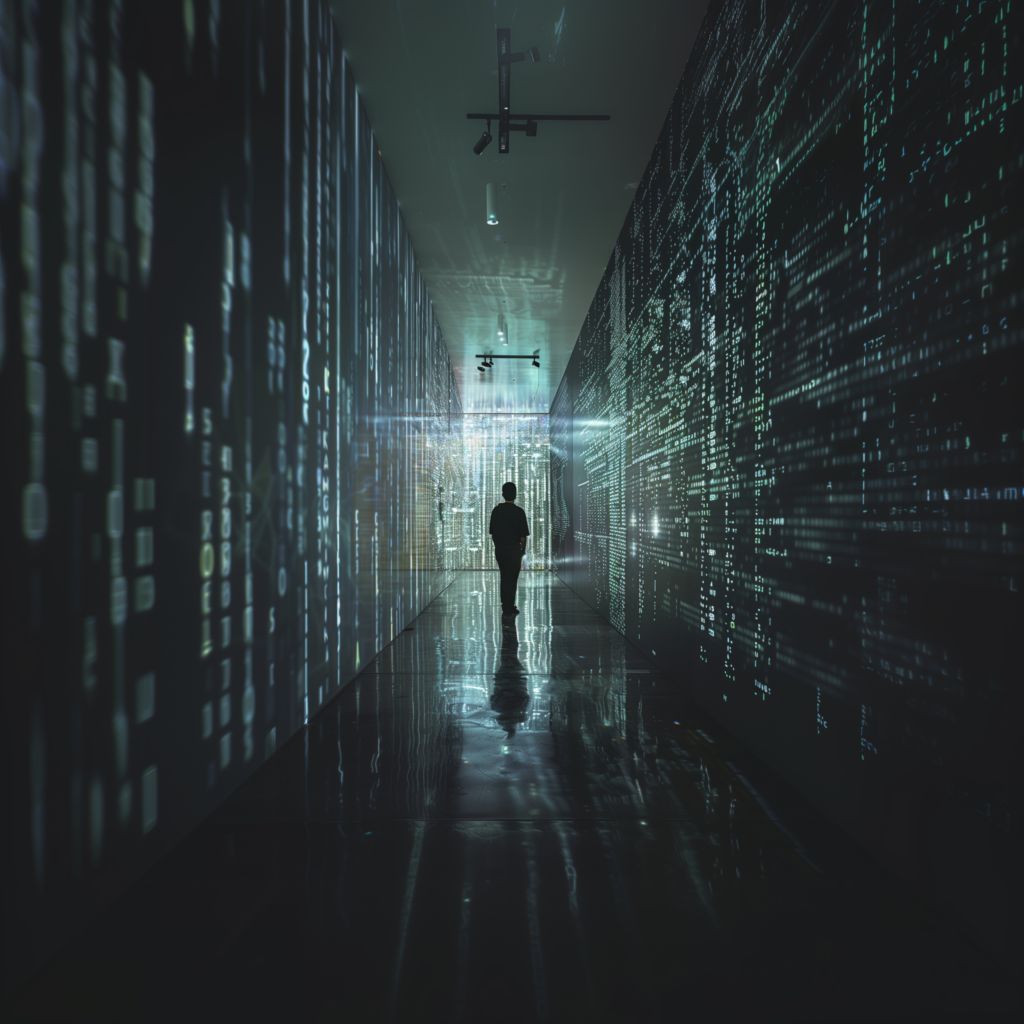
Robust Database Backup Recovery at Uber
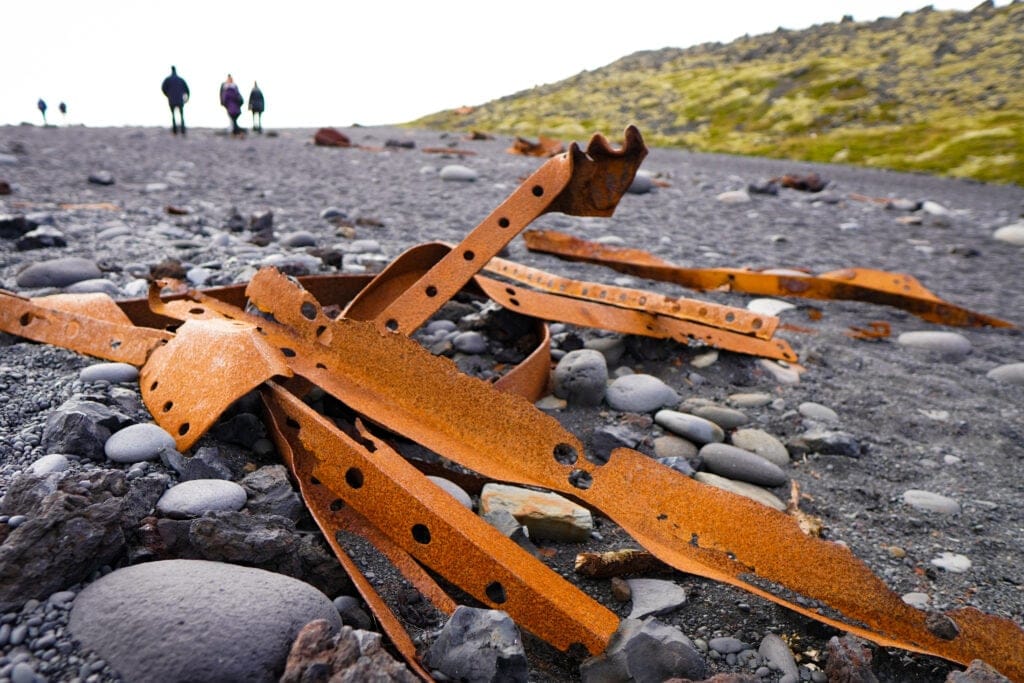