Enhanced POET: Open-Ended Reinforcement Learning through Unbounded Invention of Learning Challenges and their Solutions
May 6, 2020 / Global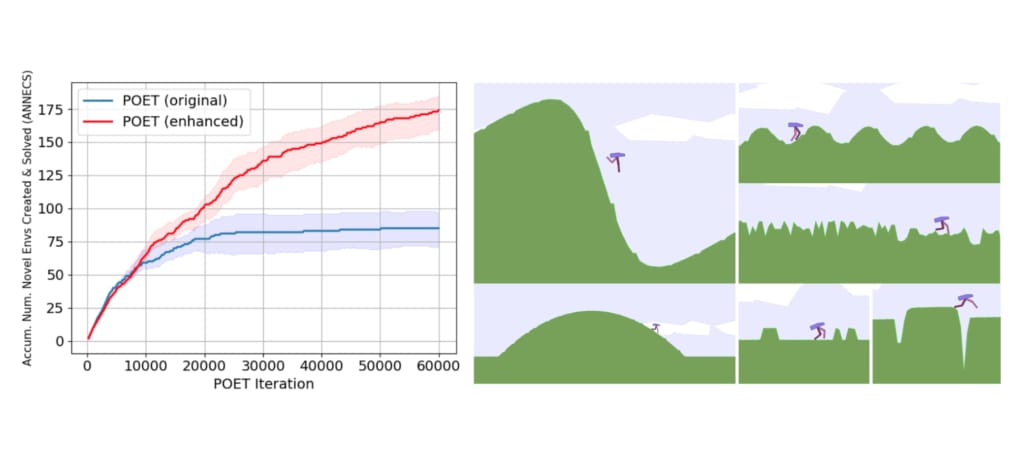
Jeff Clune and Kenneth Stanley were co-senior authors on this work and our associated research paper.
Machine learning (ML) powers many technologies and services that underpin Uber’s platforms, and we invest in advancing fundamental ML research and engaging with the broader ML community through publications and open source projects. Last year we introduced the Paired Open-Ended Trailblazer (POET) to explore the idea of open-ended algorithms. Now, we refine that project further under the name Enhanced POET, allowing for more diverse environments, incorporating an improved algorithm, and introducing a new metrics system to measure progress.
While progress in ML is often achieved through static, hand-designed benchmarks, ideally the machine could not only learn to solve benchmarks, but also to invent the right curriculum of benchmarks themselves to propel ML forward. That is why we are interested in open-ended algorithms: they offer the possibility of generating never-ending streams of novel learning opportunities and training data that could help to automate and accelerate progress in ML.
Open-endedness is fundamentally different from conventional ML, where challenges and benchmarks are often manually designed and remain static once implemented (e.g., MNIST and ImageNet for image classification, or Go for reinforcement learning). In the conventional paradigm, after a challenge or a task is solved, there is nothing to gain by running the algorithm longer in that domain.
By contrast, open-ended algorithms continually propel themselves forward by simultaneously conceiving both challenges and solutions in an autonomous and endless fashion, building their own diverse and expanding (and sometimes circuitous) curricula. Along the way, problems invented by the system (and their solutions) serve as stepping stones towards discovering and solving ever more complex and challenging problems that cannot be solved otherwise.
Such algorithms need not rely on human intuition to determine what kind of stepping stones should be included in the curricula or in what order they should be traversed. Both aspects can be discovered in parallel and asynchronously in an ever-expanding tree of diverse challenges and corresponding solutions. Ultimately, the endless creativity of such open-ended frameworks could become stepping stones towards AI-generating algorithms (AI-GAs) that could bootstrap themselves from simple beginnings to powerful problem solvers.
POET arrives
A recent advance in our efforts to develop an open-ended algorithm at Uber AI was the Paired Open-Ended Trailblazer (POET), which represents a novel path to open-endedness: It combines the advantages of several recent ideas in divergent search and population-based methods to facilitate an open-ended process of discovery within a single run.
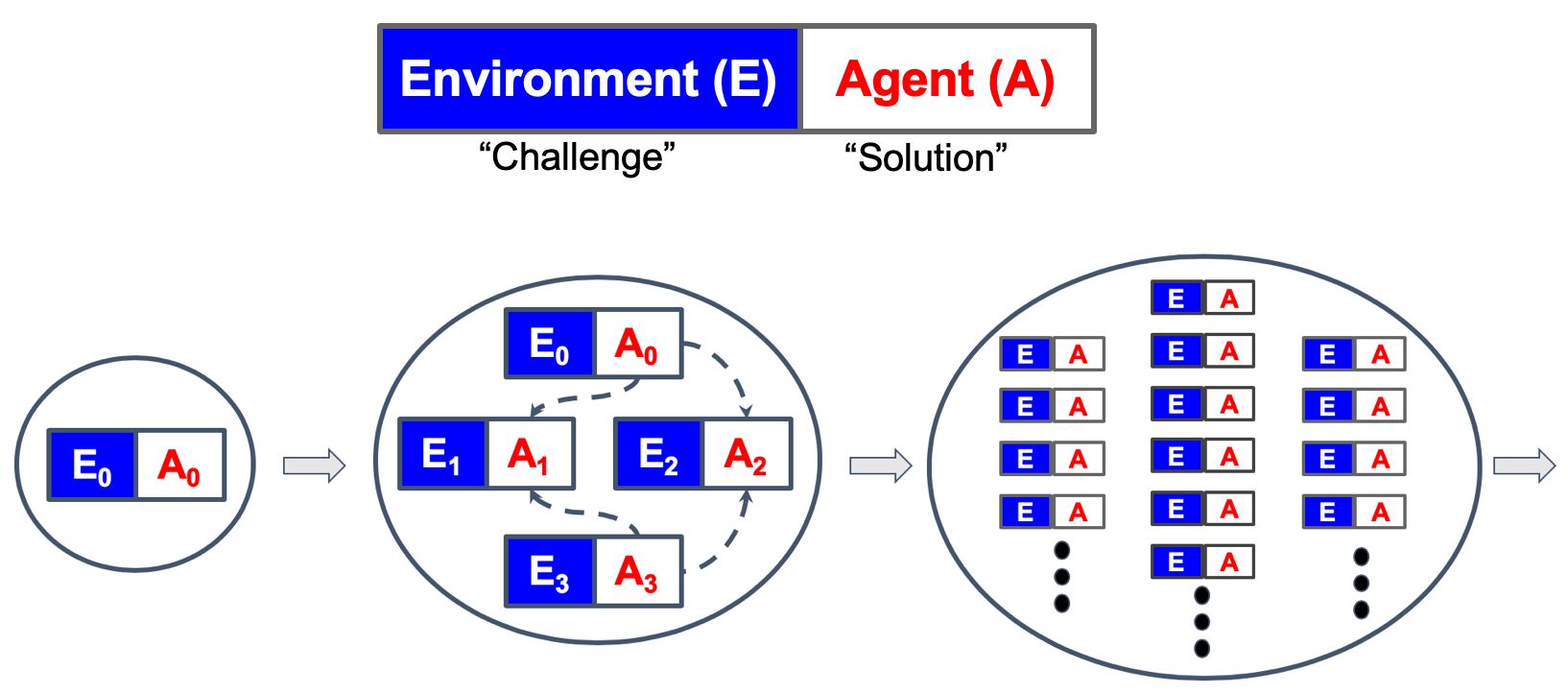
Illustrated in Figure 1, above, POET maintains and grows a population of environment-agent pairs, where each agent is optimized to solve its paired environment. POET typically starts with a trivial environment and periodically generates new environments by applying mutations (i.e. random perturbations) to the encodings of currently active environments. The encoding of an environment is a mapping from a parameter vector to an instance of an environment, creating an environmental search space (the space of all parameter vectors) that allows new realizations of the environment to be discovered.
Once generated, new environments are filtered by a minimal criterion that ensures that they are neither too hard nor too easy for existing agents in the current population, i.e. that they are likely to provide a promising environment for learning progress. From those that meet this minimal criterion, only the most novel ones are added to the population, which pushes the environments towards capturing meaningfully diverse learning opportunities.
POET also periodically checks for goal-switching opportunities: if another agent outperforms the incumbent of an environment then the incumbent is replaced (dashed arrows in Figure 1, above, represent checking for such situations), allowing innovations created to solve one problem to help solve others as well. Goal-switching essentially creates multiple, simultaneous, overlapping curricula that help POET avoid local optima and solve challenging environments that could not be solved by direct optimization or hand-designed curricula.
While POET lays a solid foundation to run an open-ended computation, the original demonstration (referred to as Original POET from here onward) of its creative potential was confined within a proof-of-concept domain of obstacle courses (modified from OpenAI Gym) with a small number of hand-picked, repeated obstacle types (e.g., stumps, gaps, and rough surfaces), which fundamentally limited the search space POET could explore.
This new work aims to unlock and demonstrate the full potential of POET. We’ve introduced several innovations that surpass the limitations of original POET, resulting in the most open-ended algorithmic demonstration to date. These innovations include:
-
- A more open-ended domain: A more expressive environmental encoding creates more open-ended and diverse environments than those in the proof-of-concept domain of Original POET.
- Enhancements to the POET algorithm: A new domain-general formulation (that works with any encoding mechanism) calculates the distance between environments, which POET harnesses to encourage the production of novel environments. We’ve also improved the goal-switching mechanism for better computational efficiency.
- A measure of progress: A novel domain-general metric called ANNECS (accumulated number of novel environments created and solved) monitors and measures the creative progress of open-ended systems including POET.
Each of these innovations is explained in more detail in the article’s following sections.
A more open-ended domain through a more expressive environmental encoding
In Original POET, the encoding of the 2D bipedal walking environments are by definition limited and intrinsically capped: the small set of hand-picked encoding parameters (e.g., ranges of stump height, gap width, and surface roughness) can only support a finite number of obstacle types with predefined regular shapes and limited variations. To create a more open-ended domain for POET to explore, we adopt a class of neural networks known as compositional pattern-producing networks (CPPNs) as a more flexible and expressive way to encode and create environmental challenges.
Illustrated in Figure 2 below, CPPNs create 2D bipedal walking environments by outputting a height y for each x-point of the landscape. Because CPPNs are typically mutated by neuroevolution algorithms such as NEAT and thus can grow to arbitrarily complex architectures, they could in theory express any possible landscape at any conceivable resolution or size, significantly expanding the breadth of possibilities of the 2D bipedal walking environments.
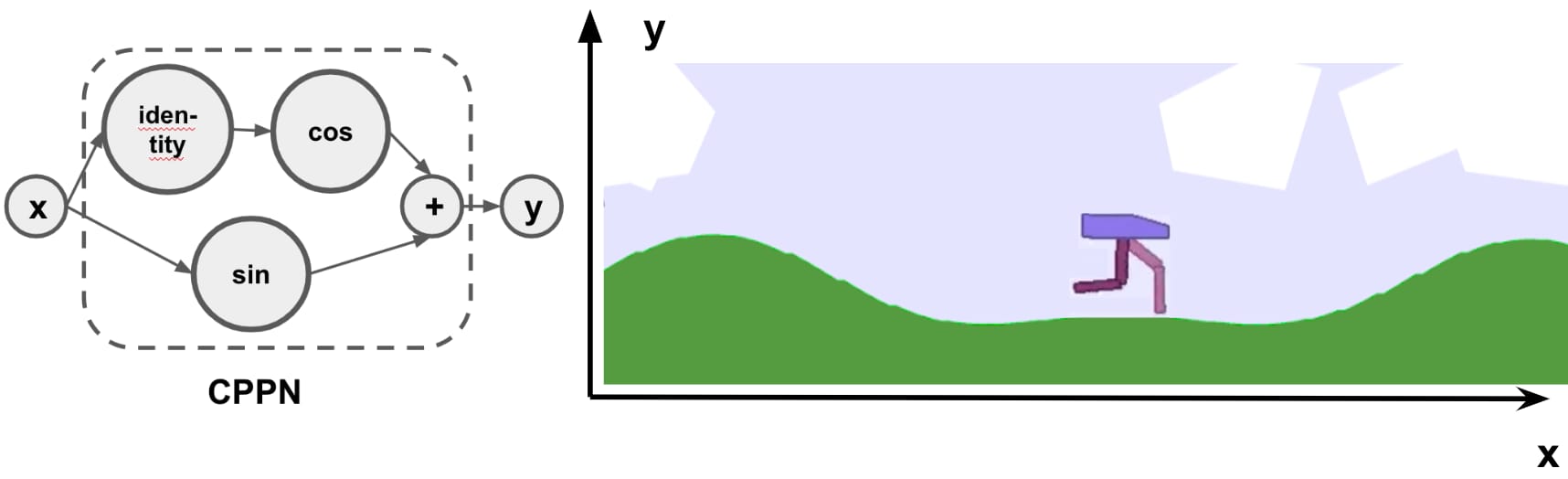
Enhancing POET: A domain-general environmental characterization and better transfer mechanism
While adopting the CPPN-based environmental encoding opens the door to more open-ended discovery by POET, it raises one question: how can we calculate the distance between environments encoded by CPPNs or, more generally, by any encoding mechanism? This question is fundamental to the success of POET, as it relies on measuring such distances to encourage the production of novel environments. The larger the average distance between a given environment and its neighbors, the more novel the environment.
In Original POET, the distance between environments is simply the distance between their encoding vectors, as the encoding was a direct, explicit description of the environment itself (e.g., the roughness of the terrain, the range of stump heights, and the range of gap sizes). The problem is that such direct mappings between the environments and their encodings no longer exist when we move to more flexible and expressive encoding mechanisms like CPPNs.
We work around this problem by formulating a domain-general environmental characterization (EC) to capture the distinctive nature or characteristics of environments in the new POET system while being completely independent of any encoding mechanism adopted. We name our proposed environmental characterization the Performance of All Transferred Agents EC (PATA-EC) because it is solely grounded by the performance (here, specifically the performance rankings) of how well all the current agents in the population perform when tested within the candidate environment rather than any domain-specific information. The underlying intuition is simple: if any newly-generated environment produces a never-seen-before rank order of how all the agents perform within it (relative to other environments), that environment likely poses a qualitatively new kind of challenge. For example, Figure 3, below, depicts how the emergence of a new landscape with bumps induces a new ordering on the three example agents, because agents with different walking gaits may differ in their ability to step over such obstacles.
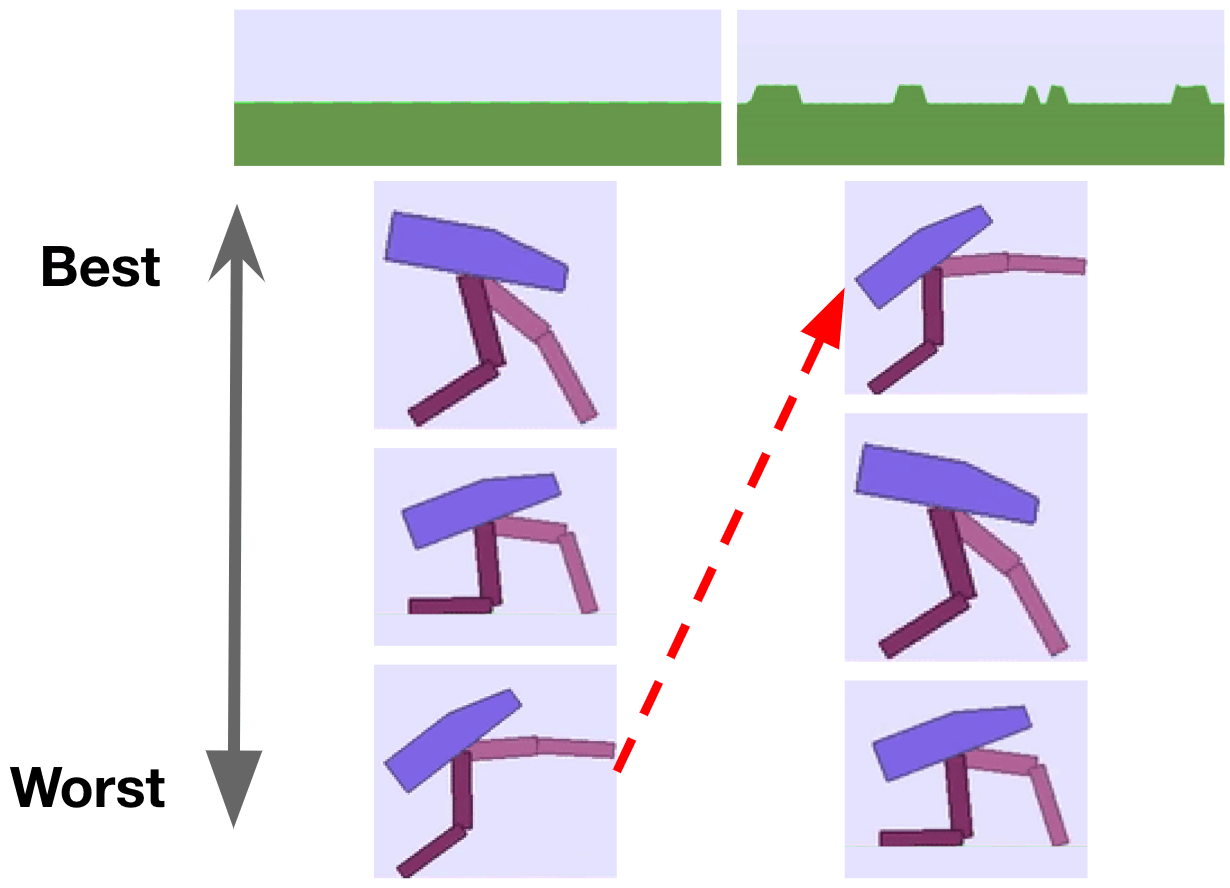
The introduction of CPPN encoding and PATA-EC enables POET to explore a much richer and more open-ended search space and potentially innovate for longer if given enough computation.
In addition, we improve the efficiency of the Original POET transfer mechanism and empirically document those efficiency gains, as described in detail in our accompanying paper.
ANNECS: A new way to measure progress in open-ended systems
Quantifying the performance of open-ended algorithms has remained elusive for the field. As there is no a priori expected outcome against which progress can be measured, how can we tell whether an open-ended system continues to generate interesting new challenges?
For a system like POET where problems coevolve indefinitely with their solutions, we propose to track—as a measure of progress—the accumulated number of novel environments created and solved (or ANNECS) from the start of the run. More concretely, for any newly created environment to be counted by ANNECS, it has to (1) pass a minimal criterion (i.e., that it is neither too hard nor too easy) measured against all the agents generated over the entire current run up to that iteration, and (2) eventually be solved by the system. In effect, these criteria ensure that we count environments that present a novel challenge to previous agents, but denies credit for generating impossible scenarios.
Our proposed metric ties directly to the overall effectiveness of an open-ended process: As the run proceeds, ANNECS consistently going up indicates that the underlying algorithm is continually creating meaningfully novel environments.
Enhanced Poet results
Enhanced POET with the new CPPN-based environmental encoding is able to create and solve a large diversity of environments within a single run—environments that are qualitatively different than those produced by the Original POET. Compare those from Original POET in Figure 4a to those produced by Enhanced POET in Figure 4b, below. (More examples are included in our accompanying paper.)
The CPPN-encoded environments produced by Enhanced POET exhibit a wide variety of obstacles that differ significantly in their overall shapes, heights, and fine details. Their paired agents demonstrate diverse skills such as traversing extreme irregularity underfoot (reminiscent of dried lava flows near volcanoes) or bracing for landing after a fall from great heights. If this piques your interest, be sure to check out videos of example Enhanced POET agents on the Uber AI YouTube channel.
![]() |
![]() |
![]() |
(a) Sample environments (and their paired agents) generated by Original POET.
![]() | ![]() |
![]() | ![]() |
![]() | |
![]() | ![]() |
(b) Sample environments (and their paired agents) generated by Enhanced POET.
Figures 4a and 4b. A comparison of sample environments from (a) the simple, hand-designed encoding in the Original POET and (b) CPPN-encoded environments created and solved by Enhanced POET. |
Such diversity is also reflected in the phylogenetic tree of the environments Enhanced POET creates in each run, which exhibit a clear signature of open-ended algorithms: multiple deep, hierarchically nested branches, resembling those from natural phylogenies, as depicted in Figure 5, below:
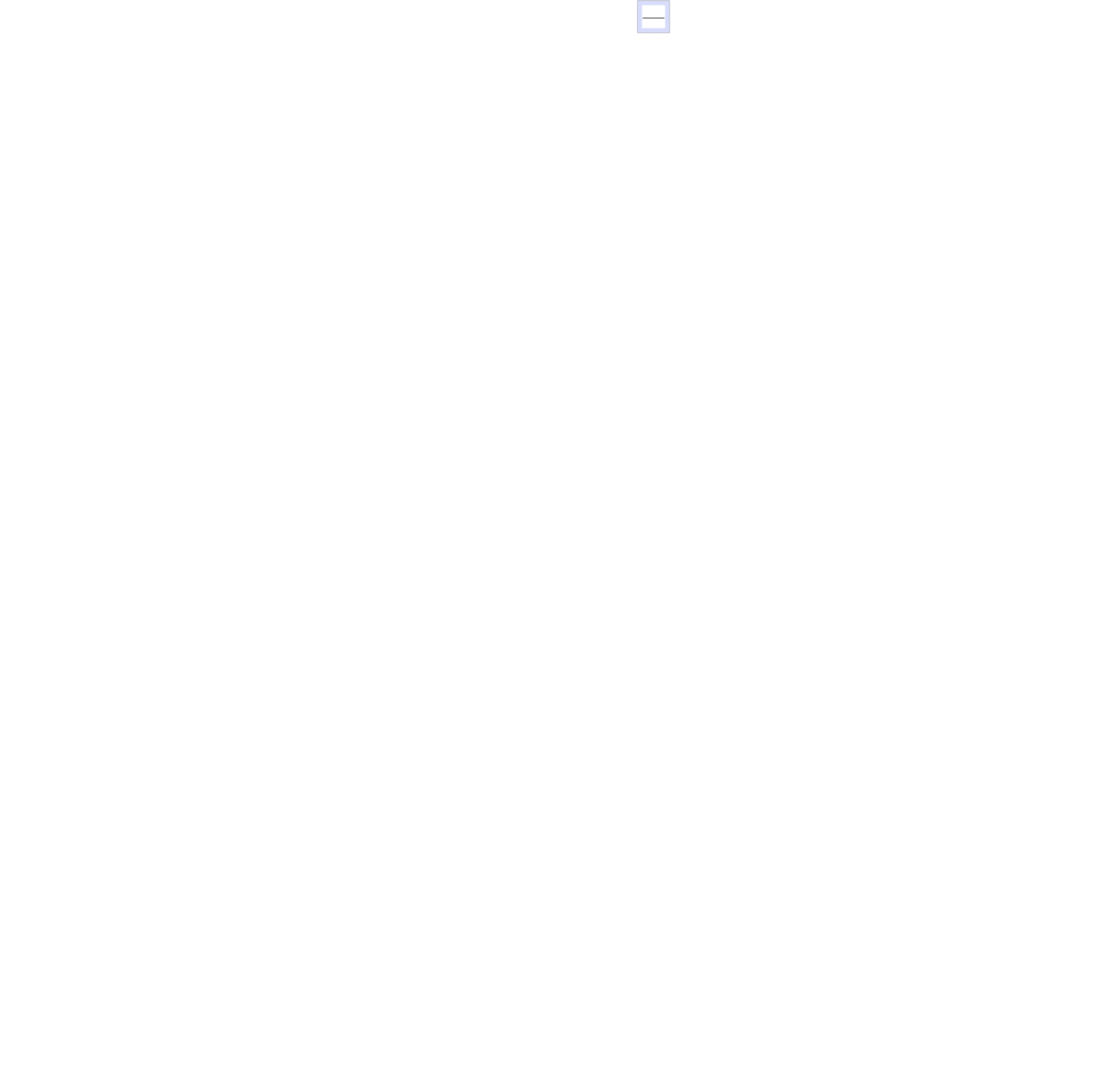
Figure 5. POET builds an ever-expanding tree of diverse environments in each run. Each node represents a unique environmental challenge that POET invented. Within each node, the overall shape of each 2D obstacle course is depicted by a (very small) line plot. For a high-resolution version, refer to Figure 10 of our accompanying paper. In the animation, the tree grows from its root in the order environments were added over the course of an Enhanced POET run.
After automatically generating these learning challenges and their solutions, our last step is to apply ANNECS (the new metric of progress) to evaluate the original and enhanced POET algorithms, as seen in Figure 6, below:
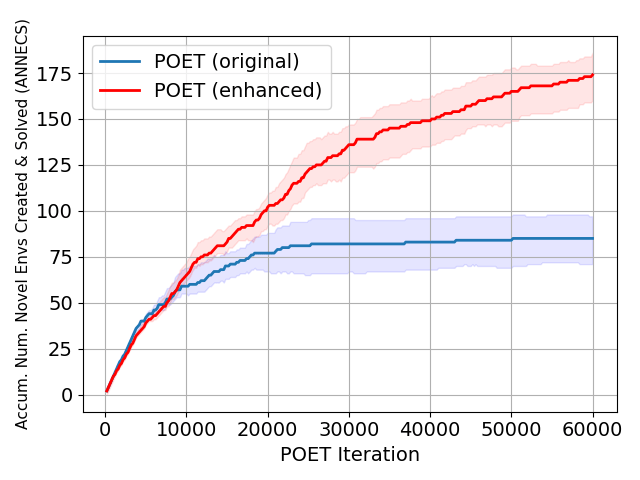
While the Original POET gradually loses its ability to create interesting new challenges, as shown by its ANNECS curve plateauing after 20,000 iterations, Enhanced POET maintains its momentum and accordingly its ANNECS value consistently increases throughout.
This result aligns with our expectation that the limited environmental encoding in Original POET cannot support long-term innovation. Enhanced POET, on the other hand, sustains innovation for longer because the more expressive and open-ended CPPN-based encoding in this work can support significantly more meaningful environmental diversity, and because the domain-general PATA-EC and improved transfer strategy make it possible and efficient to explore such a space.
The promise of open-endedness
One other particularly compelling result shown in our paper helps illustrate why open-endedness is so critical. It turns out that the very same learning algorithm performs very differently when it is embedded within POET versus when it is not. In particular, the algorithm that optimizes the agent neural networks in the above POET experiments is called evolution strategies, or ES (although other RL algorithms can also work with POET).
Interestingly, if ES is tasked on its own with solving one of the environmental challenges that POET solves, it almost always fails. In fact, it even fails if ES is combined with a linear curriculum designed to ease the way to solving the challenge. However, strikingly, ES obviously can solve these challenges because it is the optimization algorithm in POET that solved them! It’s just that it only solves them when it’s part of an overarching open-ended process.
The general lesson is that optimization algorithms may only be able to solve some hard problems when embedded within something like POET’s open-ended, divergent search process. This result was initially demonstrated in the Original POET paper, and we reconfirmed it with Enhanced POET, where its implications are more significant because of the much larger environment space.
The idea that an algorithm can only reach its potential when embedded in a process without a clear objective rests on the insight that we cannot know in advance the stepping stones that must be traversed in order to reach a far-off achievement. Original POET overcame this impediment by collecting stepping stones from innumerable divergent branching paths through the search space, many climbing towards higher complexity. While the Original POET laid a foundation for open-ended search, the Enhanced POET algorithm paves the way for us to continue to push the boundaries of open-ended algorithms and explore the potential of open-endedness. If we can conceive a domain without bounds, or at least with bounds beyond our understanding, we may one day build on the ideas in Enhanced POET to achieve something far beyond our imagination. That is the exciting promise of open-endedness.
We hope others will join us on this journey. Towards that end, we are not only releasing a paper with full technical details, but also have open sourced the code for Enhanced POET.
Acknowledgements
We thank several members of Uber AI, in particular Joost Huizinga, Thomas Miconi, Paul Szerlip, Lawrence Murray, and Jane Hung for helpful discussions. We are grateful to Leon Rosenshein, Joel Snow, Thaxton Beesley, the Colorado Data Center Team, and the entire Opus Team at Uber for providing our computing platform and for technical support.
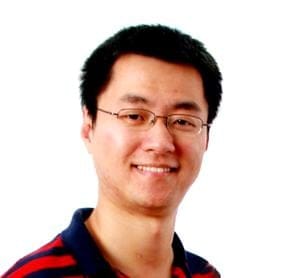
Rui Wang
Rui Wang is a senior research scientist with Uber AI. He is passionate about advancing the state of the art of machine learning and AI, and connecting cutting-edge advances to the broader business and products at Uber. His recent work at Uber was published on leading international conferences in machine learning and AI (ICML, IJCAI, GECCO, etc.), won a Best Paper Award at GECCO 2019, and was covered by technology media such as Science, Wired, VentureBeat, and Quanta Magazine.
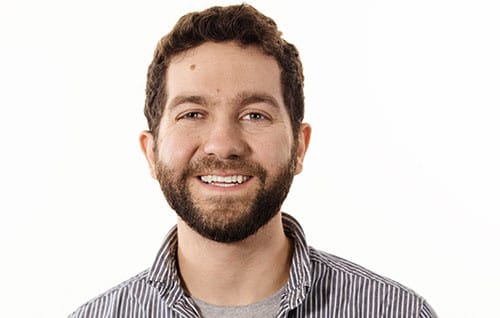
Joel Lehman
Joel Lehman was previously an assistant professor at the IT University of Copenhagen, and researches neural networks, evolutionary algorithms, and reinforcement learning.
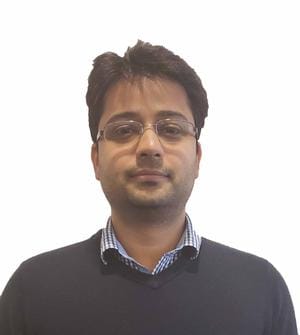
Aditya Rawal
Aditya Rawal is a research scientist at Uber AI Labs. His interests lies at the convergence of two research fields - neuroevolution and deep learning. His belief is that evolutionary search can replace human ingenuity in creating next generation of deep networks. Previously, Aditya received his MS/PhD in Computer Science from University of Texas at Austin, advised by Prof. Risto Miikkulainen. During his PhD, he developed neuroevolution algorithms to evolve recurrent architectures for sequence-prediction problems and construct multi-agent systems that cooperate, compete and communicate.
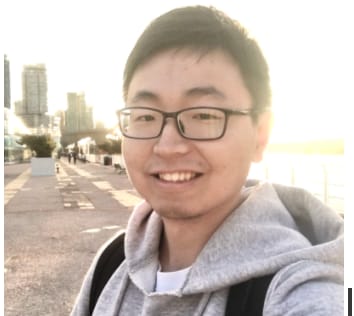
Jiale Zhi
Jiale Zhi is a senior software engineer with Uber AI. His area of interest is distributed computing, big data, scientific computation, evolutionary computing, and reinforcement learning. He is also interested in real-world applications of machine learning in traditional software engineering. He is the creator of the Fiber project, a scalable, distributed framework for large scale parallel computation applications. Before Uber AI, he was a Tech Lead in Uber's edge team, which manages Uber's global mobile network traffic and routing.
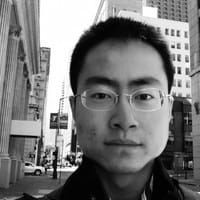
Yulun Li
Yulun Li previously worked as a software engineer with Uber AI.
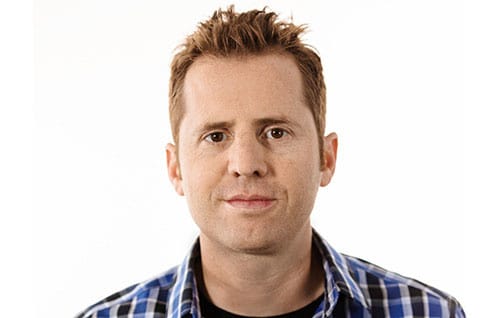
Jeff Clune
Jeff Clune is the former Loy and Edith Harris Associate Professor in Computer Science at the University of Wyoming, a Senior Research Manager and founding member of Uber AI Labs, and currently a Research Team Leader at OpenAI. Jeff focuses on robotics and training neural networks via deep learning and deep reinforcement learning. He has also researched open questions in evolutionary biology using computational models of evolution, including studying the evolutionary origins of modularity, hierarchy, and evolvability. Prior to becoming a professor, he was a Research Scientist at Cornell University, received a PhD in computer science and an MA in philosophy from Michigan State University, and received a BA in philosophy from the University of Michigan. More about Jeff’s research can be found at JeffClune.com
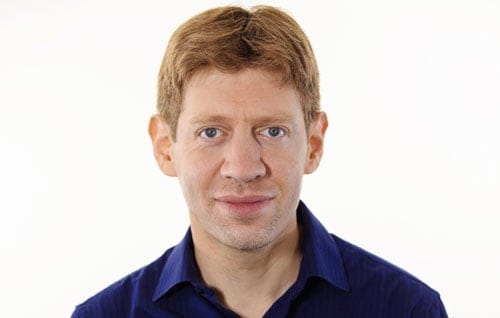
Kenneth O. Stanley
Before joining Uber AI Labs full time, Ken was an associate professor of computer science at the University of Central Florida (he is currently on leave). He is a leader in neuroevolution (combining neural networks with evolutionary techniques), where he helped invent prominent algorithms such as NEAT, CPPNs, HyperNEAT, and novelty search. His ideas have also reached a broader audience through the recent popular science book, Why Greatness Cannot Be Planned: The Myth of the Objective.
Posted by Rui Wang, Joel Lehman, Aditya Rawal, Jiale Zhi, Yulun Li, Jeff Clune, Kenneth O. Stanley
Related articles
Most popular
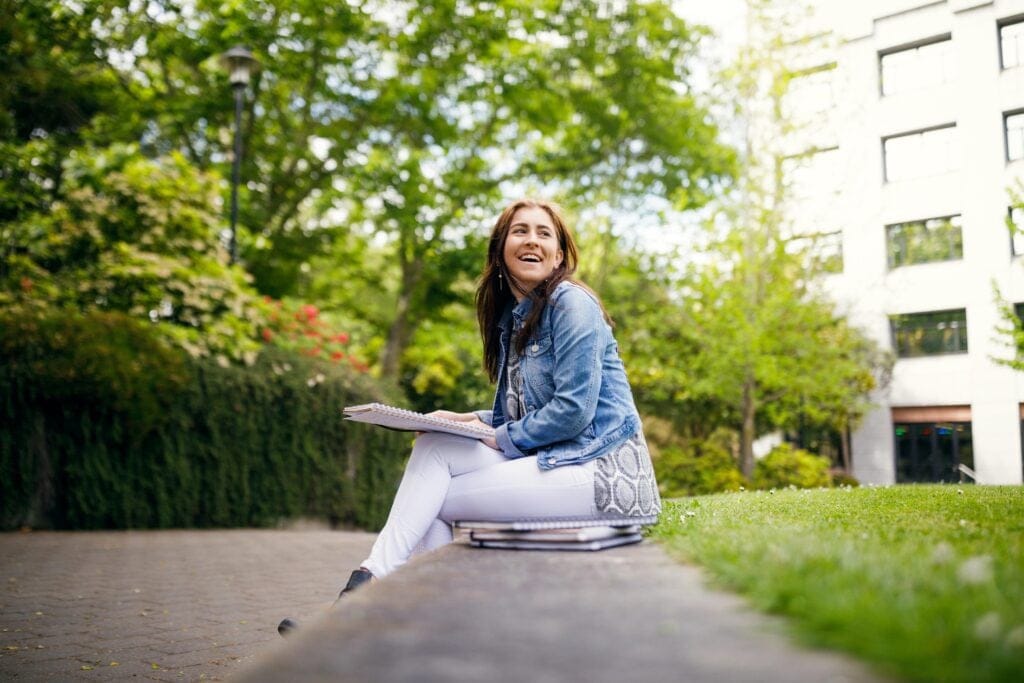
Case study: how the University of Kentucky transformed Wildcab with Uber
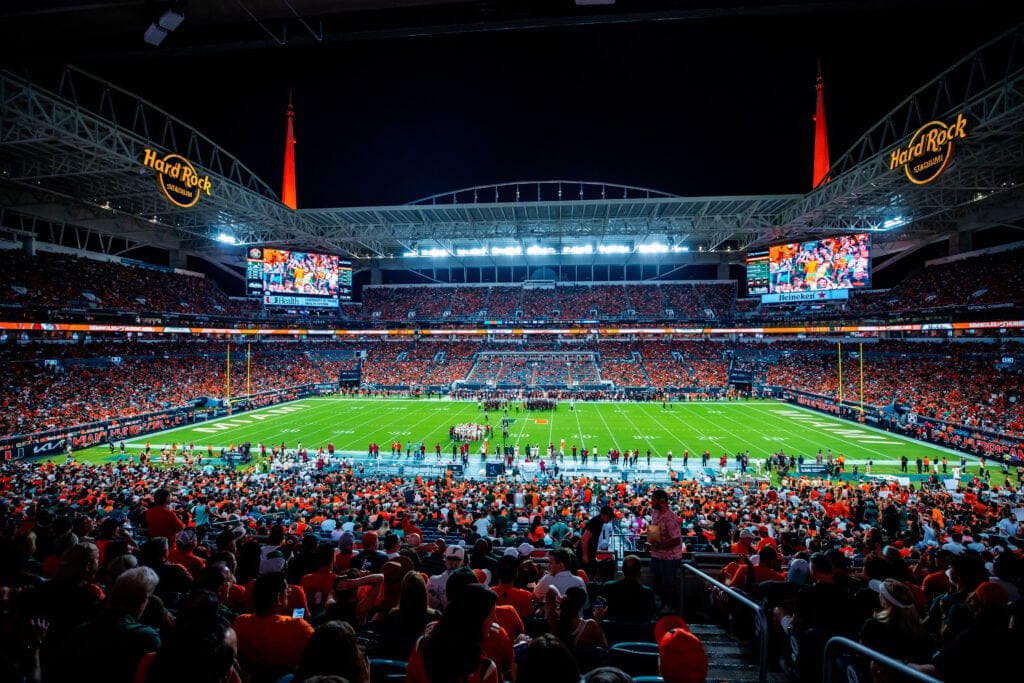
How Uber Eats fuels the University of Miami Hurricanes off the field
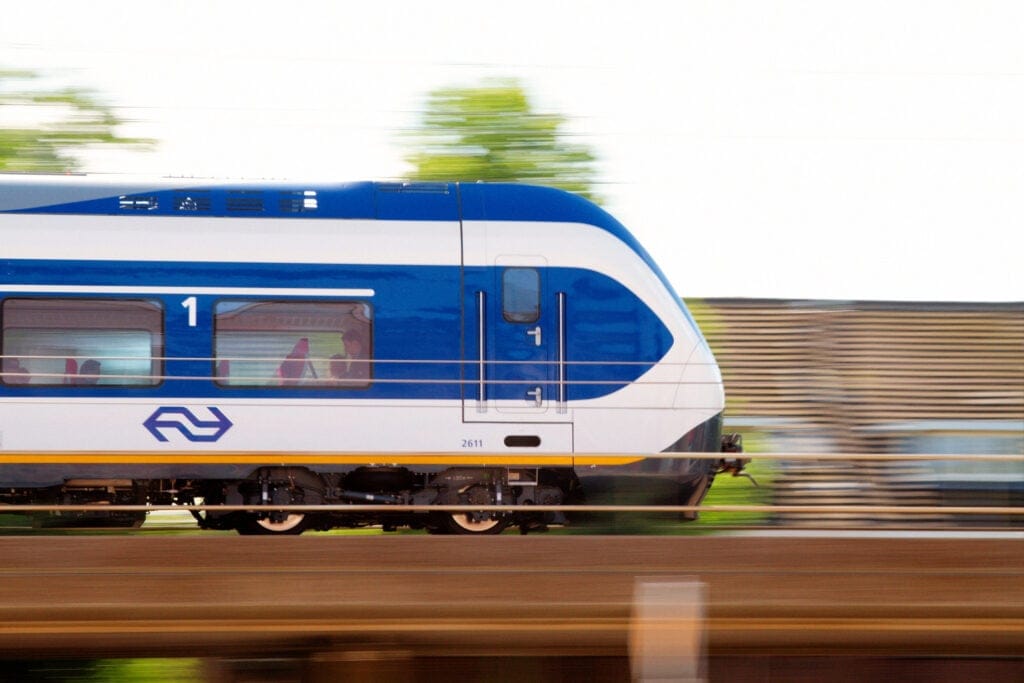
How Uber Uses Ray® to Optimize the Rides Business
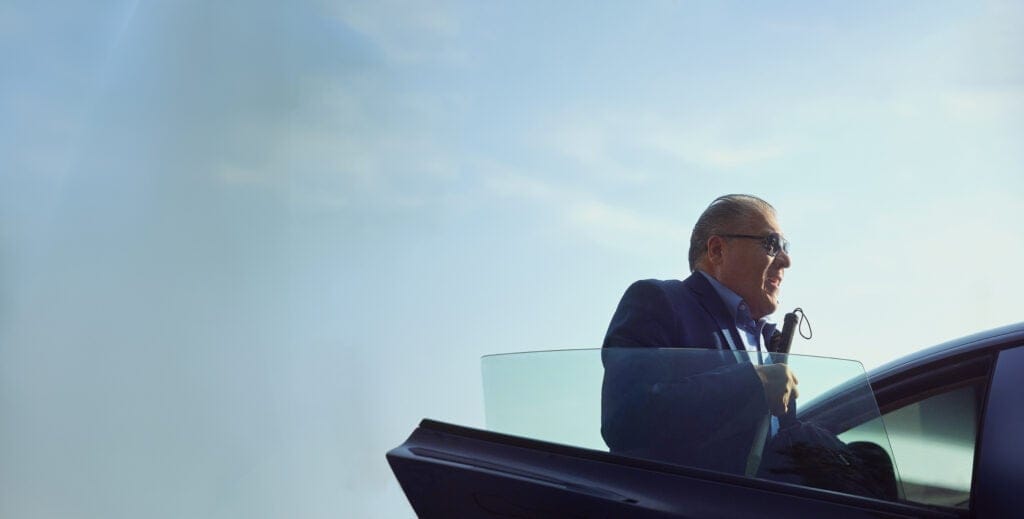
Horacio’s story: gaining mobility independence through innovative transportation solutions
Products
Company